
- This event has passed.
ESE PhD Dissertation Defense: “Balancing Fit and Complexity in Learned Representations”
July 9, 2021 at 9:00 AM - 10:00 AM
Thesis Title: Balancing Fit and Complexity in Learned Representations
Abstract: This dissertation is about learning representations of functions while restricting complexity. In machine learning, maximizing the fit and minimizing the complexity are two conflicting objectives. Common approaches to this problem involve solving a regularized empirical minimization problem, with a complexity measure regularizer and a regularizing parameter that controls the trade-off between the two objectives. The regularizing parameter has to be tuned by repeatedly solving the problem and does not have a straightforward interpretation. This work formulates the problem as a minimization of the complexity measure subject to the fit constraints.
The issue of complexity is tackled in reproducing kernel Hilbert spaces (RKHSs) by introducing a novel integral representation of a family of RKHSs that allows arbitrarily placed kernels of different widths. The functional estimation problem is then written as a sparse functional problem, which despite being non-convex and infinite-dimensional can be solved in the dual domain. This problem achieves representations of lower complexity than traditional methods because it searches over a family of RKHS rather than a subspace of a single RKHS.
The integral representation is used in a federated classification setting, in which a global model is trained from a federation of agents. This is possible due to the observation that the dual optimal variables give information about the samples which are fundamental to the classification. Each agent, therefore, learns a local model and sends only the fundamental samples over the network. This creates a federated learning method that requires only one network communication. Its solution is proven to asymptotically converges to that of traditional classification.
Next, a theory for constraint specification is established. An optimization problem with a constraint for each sample point can easily become infeasible if the constraints are too tight. In contrast, relaxing all constraints can cause the solution to not fit the data well. The constrained specification method relaxes the constraints until the marginal cost of changing a constraint is equal to the marginal complexity measure. This problem is proven to be feasible and solvable, and shown empirically to be resilient to outliers and corrupted training data.
For Zoom link, please email Elizabeth Kopeczky at: kopeczky@seas.upenn.edu.
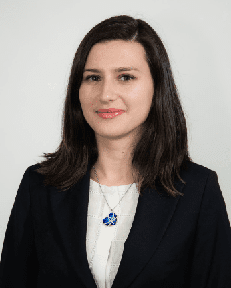
Maria Peifer
Maria Peifer received her B.Sc. degrees in electrical engineering and computer engineering from Drexel University in 2012. She completed her M.Sc. degree in electrical engineering at Rutgers University in 2015. She is currently working towards her Ph.D. in the Department of Electrical and Systems Engineering at the University of Pennsylvania in Philadelphia, PA. Furthermore, she was awarded the National Institute of Health T32 grant between 2016 and 2018 and the Dean’s scholarship between 2007 and 2012. Her research interests include signal processing and machine learning, particularly finding sparse representations in reproducing kernel Hilbert spaces and their application to various learning settings.