
- This event has passed.
MEAM Seminar: “Control of Jump Stochastic Systems by Learning Recurrent Spatiotemporal Patterns”
March 2, 2023 at 10:00 AM - 11:30 AM
Efficient learning for stochastic control and estimation remains a topic of high interest in a variety of disciplines and there are well-known advantages and disadvantages to both model-free and model-based learning. On one hand, it is becoming increasingly more feasible to rely entirely on model-free/data-driven methods for controlling complex stochastic systems, but a well-known issue with these methods is the inefficiency of their data consumption and computation time. On the other hand, most model-based control methods are designed for a very simple class of stochastic systems, e.g., Gaussian white noise systems. In this talk, our aim is to leverage the abundance of tools and theory from mathematics on various stochastic processes to expand the capabilities of model-based methods so that model-free methods don’t need to be implemented end-to-end. We demonstrate this with the broad class of jump stochastic systems (JSSs), i.e., systems with random and repetitive jump phenomena, which are an excellent case study due to the plentiful theory that exists on various jump processes and because JSSs are highly prevalent in diverse real-world applications. The core part of this talk will focus on the development of a controller architecture called “pattern-learning for prediction” (PLP) for discrete-time/discrete-event systems (e.g., Markovian jump systems), in which we take advantage of the fact that the driving stochastic process is a sequence of random variables that occurs as repeated “patterns of interest”. We then present explicit implementations of the PLP controller architecture to two real-world applications: 1) the control of networks with dynamic topology (e.g., fault-tolerant control of a power grid susceptible to line failures), for which PLP is integrated with variations of the novel system level synthesis framework for disturbance-rejection; 2) the congestion control of vehicle traffic flow over metropolitan networks of signalized intersections, for which PLP is extended to a version called “pattern learning with memory and prediction” via the integration of episodic control to reduce memory consumption.
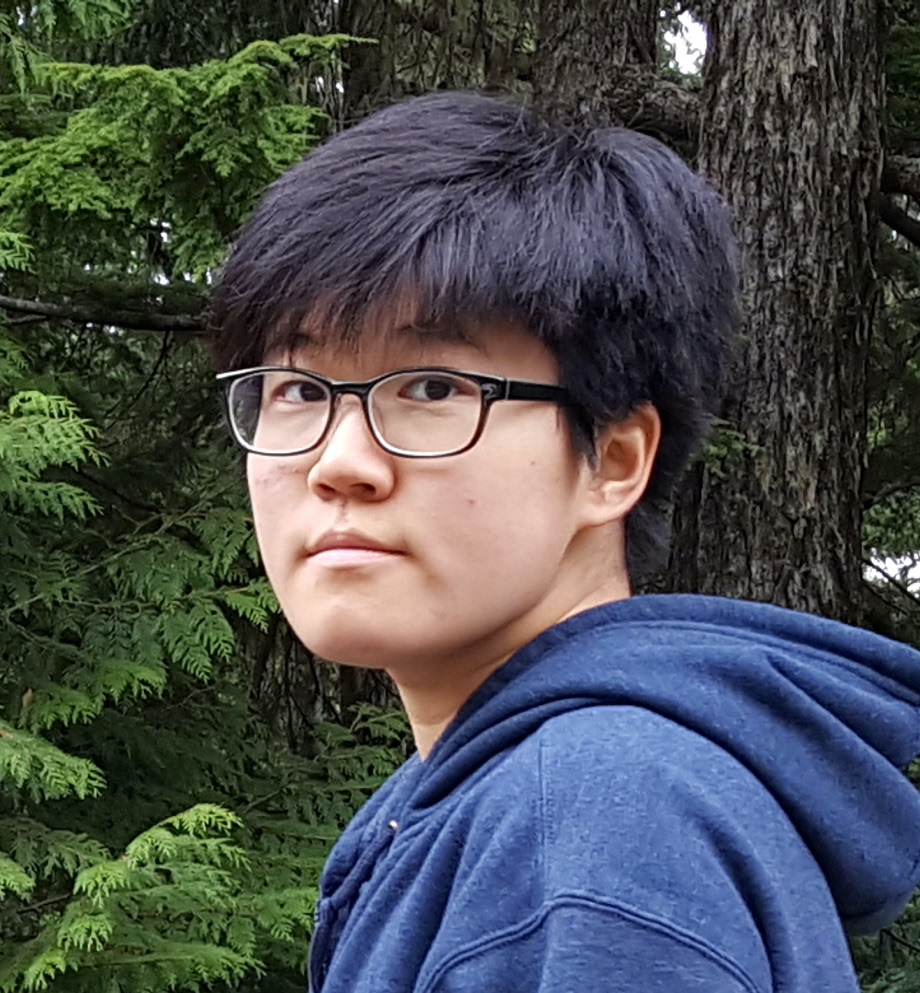
SooJean Han
Postdoctoral Scholar, Autonomous Robotics and Control Laboratory (ARCL), California Institute of Technology
SooJean Han is a postdoctoral scholar at the Autonomous Robotics and Control Laboratory (ARCL) at Caltech. She received her Ph.D. in Control and Dynamical Systems at Caltech under the supervision of Professor John C. Doyle and Professor Soon-Jo Chung in January 2023. She received her B.S. degree in Electrical Engineering and Computer Science (EECS) and Applied Mathematics at UC Berkeley in 2016. In 2017, she was a research assistant in the Hybrid Systems Lab at UC Berkeley and a research assistant in the Caltech CAST Lab. She was also an intern at JPL in 2018 as a member of Team CoSTAR for the DARPA Subterranean (subT) Challenge. She received the Caltech Special EAS Fellowship and the NSF GRFP. Her research interests lie in the efficient control for stochastic systems that have recurrent jump phenomena, with applications in cyberphysical systems, robotics, and biology, especially large-scale networks and fault-tolerance.