
- This event has passed.
ESE Thesis Defense: Cassiano Becker
November 20, 2019 at 10:00 AM - 11:30 AM
Title: “Data-Driven Modeling, Analysis and Design of Networked Dynamical Systems with Applications in Neuroscience”
Abstract: In this thesis, we provide contributions to the modeling, analysis and design of networked dynamical systems from a data-driven perspective. Our approach is grounded on the integration of concepts and tools from graph theory, control theory, optimization, and statistics. In particular, we develop methodologies that can be applied to challenging, high-dimensional problems where only partial or summarized information about the structure and function of a complex system is available. We validate our methods across different application domains, most remarkably with large-scale neuroimaging datasets that follow state-of-the-art acquisition techniques and span multiple individuals and experimental paradigms.
Structurally, the contributions of this thesis have been divided in three parts. In the first part, we address the topic of inference and analysis of complex networks. In particular, we establish data-driven models and algorithms that approximate measures of functional connectivity, derived from a system’s potentially complex dynamics, as a matrix mapping based on structural connectivity information. Using tools from spectral graph theory, we propose a mapping technique that is able to systematically account for the role of indirect structural walks in the generation of functional associations. We then apply our method to obtain accurate structural-to-functional connectivity mappings for brain networks derived from functional magnetic resonance (fMRI) and diffusion imaging measurements, and perform a series of analyses of the generated mappings in terms of their spectral characteristics.
In the second part, we devote our attention to the problem of identification of system dynamics. First, we generate data-driven dynamic models that capture the influence of cognitive inputs on brain signals that are observed through fMRI measurements. More specifically, we extend subspace system identification methods to create large-scale, linear time-invariant representations for both single and multiple-individual configurations of a motor task-fMRI experiment, and apply control-theoretic tools to analyze the dynamical characteristics of the resulting models. Subsequently, to abate some general limitations of linear time-invariant models, we propose three novel system identification approaches. Specifically, we (i) enable the identification of state-space linear time-varying models through a generalized expectation maximization method, (ii) perform the estimation of linear parameter-varying models having a latent parameter space via Bayesian variational inference, and (iii) implement blind subspace identification using Riemannian optimization.
In the last part of the thesis, we focus on the topic of network design for dynamic performance. More specifically, we address the problem of finding edge weights of a linear networked dynamical system such that certain bounds on its controllability metrics are satisfied. In particular, we consider the worst-case (i.e., minimum eigenvalue of the controllability Gramian) and average-case (i.e., trace of the inverse controllability Gramian) metrics. Algorithmically, we provide a principled convex relaxation approach that exhibits guaranteed convergence and known global optimal value. Our methodology includes the possibility to consider cost functions over the edge weights which can be used, for example, to promote solutions with higher sparsity in the required edge modifications. Finally, we verify our results with numerical simulations over many random network realizations, and with multi-agent and power system topologies.
Advisor:
Victor M. Preciado, Associate Professor of Electrical and System Engineering
Graduate Chair of Electrical and System Engineering
Department of Electrical and Systems Engineering
Department of Applied Mathematics and Computational Science
University of Pennsylvania
Committee:
George J. Pappas (chair), Full Professor and Department Chair
Department of Electrical and Systems Engineering, Department of Computer and Information Sciences, Department of Mechanical Engineering and Applied Mechanics
University of Pennsylvania
Danielle S. Bassett (member), J. Peter Skirkanich Professor
Department of Bioengineering, Department of Electrical and Systems Engineering Department of Physics and Astronomy, Department of Neurology, Department of Psychiatry
University of Pennsylvania
Sergio Pequito (member), Assistant Professor
Department of Industrial and Systems Engineering
Department of Electrical, Computer, and Systems Engineering
Rensselaer Polytechnic Institute
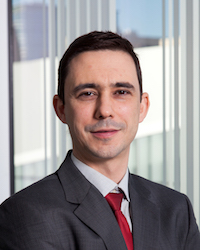
Cassiano Becker
Ph.D. Candidate, Penn Electrical and Systems Engineering Dept.
Cassiano Becker completed an M.Sc. in Telecommunications (with distinction) from University College London and a B.Eng. in Electrical Engineering from UFRGS, Brazil. Before starting his doctoral studies he worked as systems engineer, software developer and project manager in the telecommunications industry. His research interests include inference and control problems in complex networks using advanced techniques in optimization and machine learning, with applications in cyber-physical systems and neuroscience.