
- This event has passed.
CIS Seminar: “Enhancing Usability and Explainability of Data Systems”
February 24, 2021 at 3:00 PM - 4:00 PM
The recent growth of data science has expanded its reach to an ever-growing user base of non-experts. Unfortunately, most existing systems offer limited usability and support for explanations: these systems are usable only by experts with sound technical skills, and even experts are hindered by the lack of transparency into the inner workings of the systems. My research focuses on solving the challenges users face while interacting with data systems and, thus, pushes the frontiers towards achieving democratization of data systems, demanding that people with different skills and backgrounds should be able to use these data systems alike. Further, my research aims at providing explainability of the behavior of data systems to help the users understand and trust the system function, especially when unexpected behavior occurs. In this talk, I will discuss frameworks that address these challenges in a variety of settings and applications, including relational data querying, document summarization, system debugging, and trust in machine-learning models that learn from data.
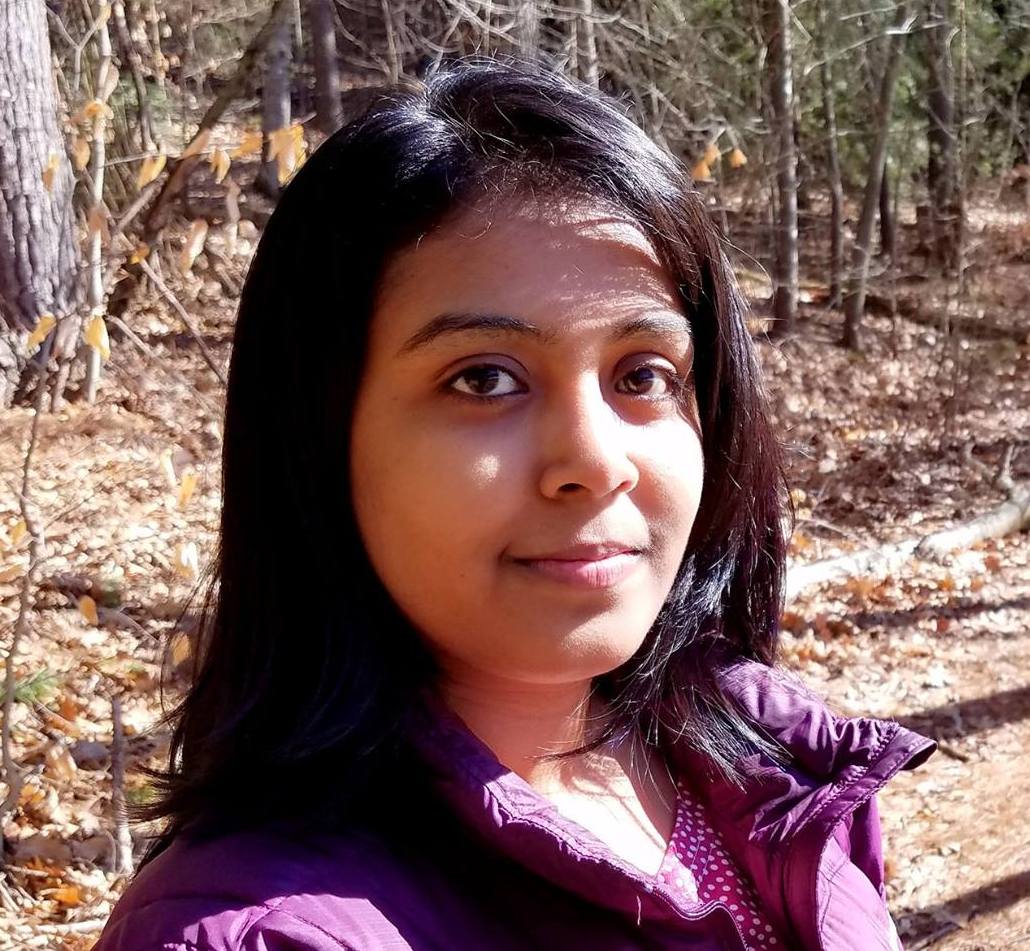
Anna Fariha is a Ph.D. candidate in the College of Information and Computer Sciences at the University of Massachusetts, Amherst, advised by Professor Alexandra Meliou. Her work is highly interdisciplinary; she has applied her research in data management to a broad range of domains of computer science, including program synthesis, software engineering, machine learning, natural language processing, and human-computer interaction. She is interested in designing mechanisms for enhancing system usability and developing intelligent tools towards boosting productivity and agility for a diverse group of users, ranging from end users to data scientists and developers. Her research has been published in top-tier data management venues, including SIGMOD and VLDB, and her work has been recognized with several awards, including a Microsoft Research Dissertation Grant and the VLDB 2020 Best Demonstration Runner-up Award.