
- This event has passed.
Spring 2025 GRASP Seminar: Robin Walters, Northeastern University, “Pushing the Limits of Equivariant Neural Networks”
April 22 at 10:00 AM - 11:00 AM
This will be a hybrid event with in-person attendance in AGH 306 and virtual attendance on Zoom.
ABSTRACT
Despite the success of deep learning, there remain challenges to progress. Deep models require vast datasets to train, can fail to generalize under surprisingly small changes in domain, and lack guarantees on performance. Incorporating symmetry constraints into neural networks has resulted in models called equivariant neural networks (ENN) which have helped address these challenges. I will discuss several successful applications, such as trajectory prediction, ocean currents forecasting, and robotic control. However, there are also limits to the effectiveness of current ENNs. In many applications where symmetry is only approximate or does apply across the entire input distribution, equivariance may not be the correct inductive bias to aid learning and may even hurt model performance. I will discuss recent work theoretically characterizing errors that can result from mismatched symmetry biases which can be used for model selection. I will also suggest different methods for relaxing symmetry constraints so that approximately equivariant models can still be used in these situations.
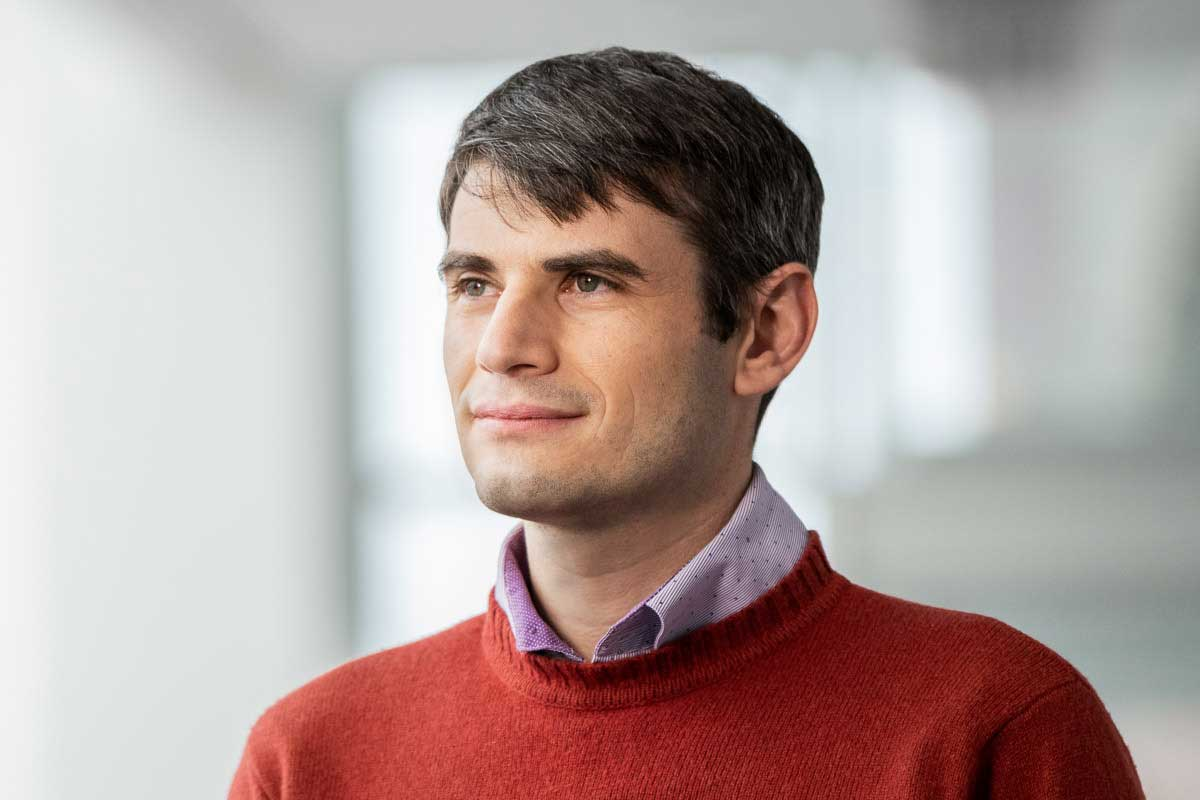
Robin Walters
Northeastern University
Robin Walters is an assistant professor in the Khoury College of Computer Sciences at Northeastern University, based in Boston.
Walters’ research focuses on the role of symmetry in deep learning. By exploring ways of building a problem’s symmetry into a deep learning model as hard mathematical constraints, Walters has discovered it’s possible to improve not just that model’s data efficiency, but its generalization and trustworthiness as well. Now, as director of the Geometric Learning Lab, Walters is pushing the limits of these methods, making use of approximate symmetry and exploring everything from the theory of underlying symmetry in neural network structure to the subject’s range of practical applications.
Walters also brings his background as a mathematician into the classroom, where he teaches computational theory. He enjoys watching his students develop the ability to think rigorously and communicate clearly about the complex topic, and he particularly enjoys mentoring graduate students. Walters is a visiting fellow at the Boston Dynamics AI Institute, where he develops equivariant neural networks for sample efficient robot perception and manipulation.