
- This event has passed.
Spring 2024 GRASP SFI: Joseph DelPreto, Massachusetts Institute of Technology, “Using Sensing and AI to Enrich Human Interactions with Machines and Nature”
March 13, 2024 at 3:00 PM - 4:00 PM
This will be a hybrid event with in-person attendance in Levine 307 and virtual attendance on Zoom.
ABSTRACT
Coupling advanced wearable and environmental sensors with dynamic AI frameworks has the potential to transform how we engage with machines and with the natural world. By co-developing intelligent sensors and deployable machine learning pipelines, we can unlock the power of data to address impactful challenges in fields ranging from human-robot collaboration to environmental science. Realizing this vision will require a multifaceted approach including networks that extract insights from large streams of continuous data, algorithms that adapt to new subjects or environments from limited examples, unobtrusive sensing with embedded autonomy, scalable multimodal dataset curation, and paradigms for fluid human interactions with AI systems. Moving towards these goals, this talk will present approaches to creating more intelligent and fluid human-robot interactions by leveraging wearable sensors for brain, muscle, and motion activity combined with adaptive learning pipelines. It will explore how these techniques can scale to curate multimodal datasets of human behavior that aim to support foundational models of physical intelligence. To help improve sensing capabilities, it will also discuss techniques for creating soft wearable sensors with embedded learning pipelines. Finally, recent results of using wearable and deployable sensors to study non-human species will be introduced; combining robust wearable sensor tags, drones, and new machine learning pipelines can reveal exciting insights into the language and culture of sperm whales.
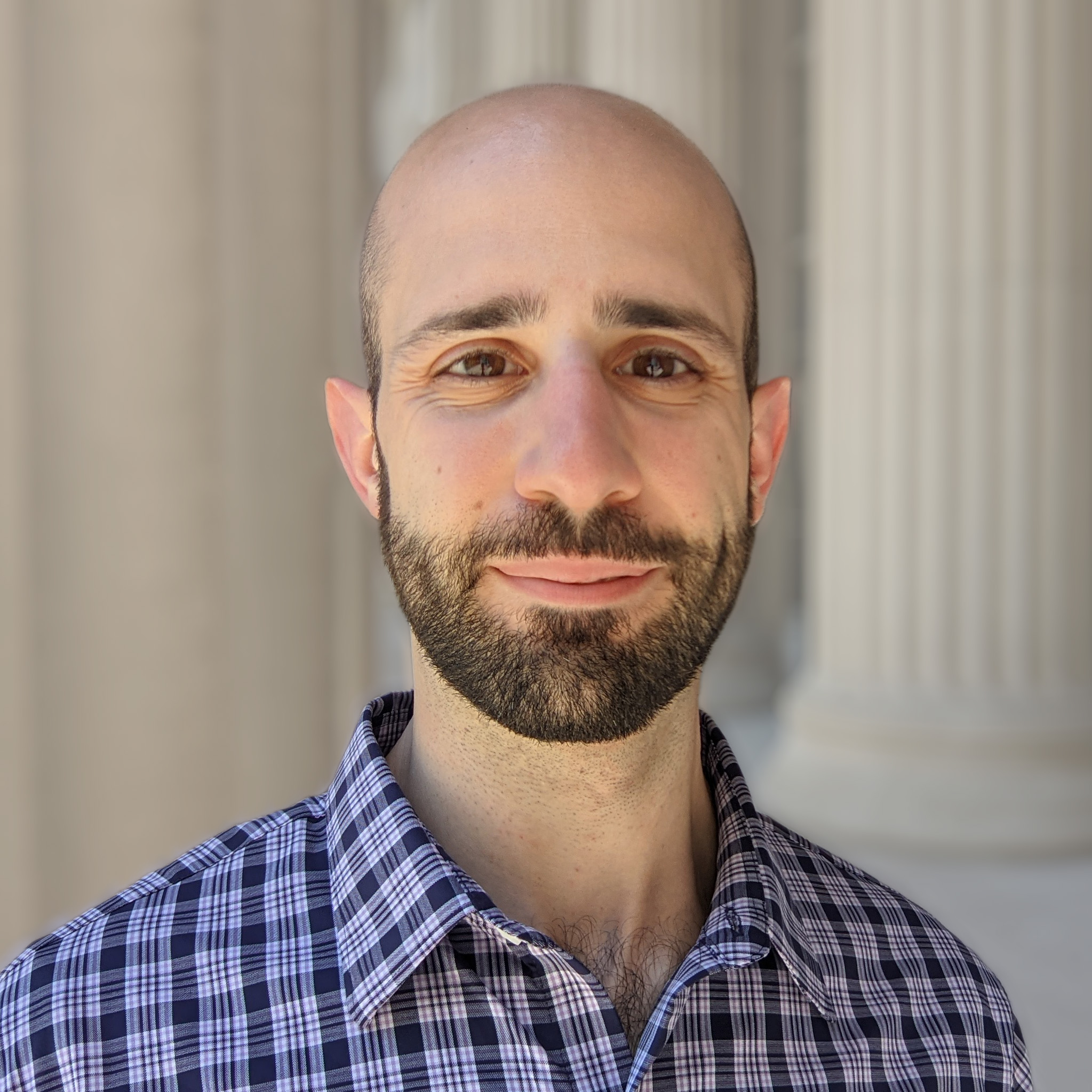
Joseph DelPreto
Massachusetts Institute of Technology
Joseph DelPreto is a Postdoctoral Associate at MIT’s Computer Science and Artificial Intelligence Lab (CSAIL) working with Daniela Rus. His research focuses on designing machine learning pipelines, devices, and datasets that improve how we interact with machines and nature. By creating smart wearables and deployable sensing that continuously curate datasets of human or animal behavior, he aims to fuel data-driven approaches for increasing productivity, quality of life, and sustainability. He has explored applications to human-robot collaboration, healthcare, and environmental science. His Ph.D. at MIT focused on using muscle signals, brain signals, and motion signals to teach, collaborate with, and supervise robot assistants. His M.S. work at MIT developed systems for generating on-demand printable robots, and included minors in finance and cognitive neuroscience. He received his B.S. from Columbia University with a major in Electrical Engineering and minors in Mechanical Engineering and Psychology.