
- This event has passed.
MSE Seminar: “Probabilistic Digital Twins for Structure Preserving Simulation and Scientific Discovery”
April 25, 2024 at 10:30 AM - 12:00 PM
Despite the recent flurry of work employing machine learning to develop surrogate models to accelerate scientific computation, the “black-box” underpinnings of current techniques fail to provide the verification and validation guarantees provided by modern finite element methods. In this talk we present a data-driven finite element exterior calculus for building accelerated reduced-order models of multiphysics systems when the governing equations are either unknown or require closure. Key to the framework is a fully differentiable partition of unity which provides a machine learnable alternative to a traditional computational mesh, upon which we simultaneously learn physical relevant control volumes alongside corresponding integral balance laws. We demonstrate that resulting models may realize speedup of over 1000x over traditional finite element simulations, while guaranteeing the exact treatment of physical constraints and numerical stability. We then briefly summarize recent work developing Bayesian underpinnings for these models, providing characterization of epistemic uncertainty which may be used to drive active learning tasks.
With tools for building probabilistic digital twins in hand, we then turn to our work integrating physical models into high-throughput material discovery experiments to characterize process-structure-property relationships. In material science, datasets are comparatively small relative to the combinatorially massive space of potential designs. We combat this by fusing information spanning multimodal characterization (e.g. XRD,TEM,SEM,EBSD) of differing fidelity and throughput and incorporating data-driven models. We end by summarizing some campaigns conducted at Sandia National Laboratories applying these tools to physical vapor deposition, metal additive manufacturing, and electrodeposition.
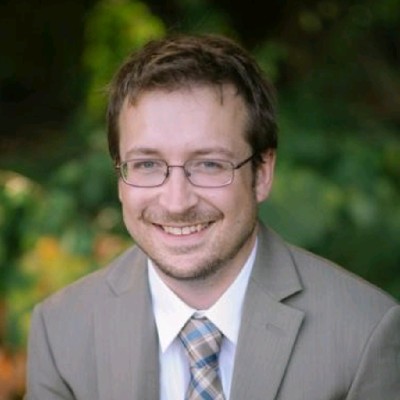
Nat Trask
Associate Professor, Department of Mechanical Engineering and Applied Mechanics, University of Pennsylvania
Nat Trask is an Associate Professor in the Mechanical Engineering and Applied Mechanics department at UPenn and holds a joint appointment with the Center for Computing Research at Sandia National Laboratories. He spent the past eight years at Sandia in Albuquerque, NM as a Principal Member of Technical staff, leading a group developing machine learning for scientific computing and for high-throughput material discovery. Prior to that he obtained his PhD in Applied Mathematics at Brown University studying with Professor Martin Maxey. His research spans a range of multiphysics and multiscale problems, including: construction of probabilistic digital twins; multimodal learning; and data-driven modeling, with applications to problems spanning fusion power, additive manufacturing, climate, semiconductor physics, shock physics, and multiscale fracture problems.