
- This event has passed.
MEAM Seminar: “Wall-modeled LES of 3-D Turbulent Boundary Layer with Emphasis on Grid Independency”
June 5, 2023 at 10:00 AM - 11:00 AM
The capability to predict high-Reynolds-number turbulent flows is essential for many natural and engineering flows such as external aerodynamics (wind turbines, aircraft wings), hydrodynamics (the hull of marine vehicles) and atmospheric boundary-layer flow over complex landscapes and cityscapes. However, due to extreme disparity of scales present in high-Reynolds-number wall-bounded turbulent flows, any attempt to simulate these flows directly without resorting to modeling of some sort results in prohibitively large computational cost. Among various modeling paradigms, wall-modeled large-eddy simulation (WMLES) has the potential to be both predictive and affordable, capturing more of the complex flow physics in the outer portion of boundary layers while modeling the expensive inner layer.
In this talk, I will first present a comparative study of WMLES of a turbulent boundary layer with mean-flow three-dimensionality developing on the floor of a bent square duct, which mimics the flow over the swept wing of the aircraft. It is demonstrated that more complex wall models better predict the near-wall three-dimensionality. The wall-stress direction from the wall models is shown to have separable contributions from the equilibrium stress part and the integrated nonequilibrium effects, where how the latter is modeled differs among the wall models. Second, I will discuss the ill-defined notion of grid convergence in WMLES. I propose that the convergence rate of WMLES is controlled by the extent of the wall-modeled region. A new perspective here is that one may attempt to converge WMLES at the desired grid resolution, while the accuracy of the converged solution can be attributed primarily to wall models. Lastly, I will talk about a new perspective on the dynamic LES models for subgrid-scale closure. I will show that there are dynamically important directions along which dynamic models can be further optimized.
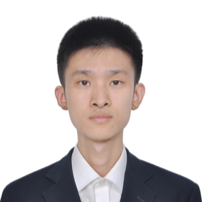
Xiaohan Hu
Ph.D. Candidate, Department of Mechanical Engineering & Applied Mechanics, University of Pennsylvania
Advisor: George I. Park