
- This event has passed.
MEAM Seminar: “Towards Personalized Predictive Human Models”
April 26, 2022 at 10:00 AM - 11:30 PM
Numerical simulation of physical phenomena is a powerful tool embraced by scientists and engineers for decades. Using simulation tools to predict human movements is particularly critical for training AI-enabled robots interacting with humans, providing testbeds for wearable device design, and generating a wealth of labeled, high-fidelity human motion data. However, existing physics simulators and motor control algorithms for modeling human movements were developed with a fictitious “average human” in mind, while in reality we are often more interested in predicting the motion of a particular real person. Pursuing the quest towards building personalized predictive human models, we develop a learnable and differentiable physics simulator to harness the power of data, and a data acquisition pipeline to provide large-scale biomechanical motion data for the learnable simulator to consume. These computational tools can potentially simulate a wide range of scenarios, but also provide the option to be personalized to specific individuals using only a moderate amount of data. While we focus on the application domains related to human movements, these tools are general and applicable to other robotic research problems on optimal control and parameter estimation. In this talk, I will describe our overall vision on perusing personalized predictive human models, as well as a collection of projects that advanced the state of the art toward this vision.
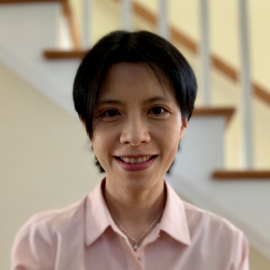
C. Karen Liu
Associate Professor, Department of Computer Science, Stanford University
C. Karen Liu is an associate professor in the Computer Science Department at Stanford University. Prior to joining Stanford, Liu was a faculty member at the School of Interactive Computing at Georgia Tech. She received her Ph.D. degree in Computer Science from the University of Washington. Liu’s research interests are in computer graphics and robotics, including physics-based animation, character animation, optimal control, reinforcement learning, and computational biomechanics. She developed computational approaches to modeling realistic and natural human movements, learning complex control policies for humanoids and assistive robots, and advancing fundamental numerical simulation and optimal control algorithms. The algorithms and software developed in her lab have fostered interdisciplinary collaboration with researchers in robotics, computer graphics, mechanical engineering, biomechanics, neuroscience, and biology. Liu received a National Science Foundation CAREER Award, an Alfred P. Sloan Fellowship, and was named Young Innovators Under 35 by Technology Review. Liu also received the ACM SIGGRAPH Significant New Researcher Award for her contribution in the field of computer graphics. In 2021, Liu was inducted to ACM SIGGRAPH Academy.