
- This event has passed.
MEAM Seminar: “Deep Learning and Uncertainty Quantification: Methodologies and Applications”
August 24, 2021 at 10:30 AM - 12:00 PM
Uncertainty is ubiquitous in physical and engineering science because of the lack of knowledge, inaccuracy in measurements, objective stochastic nature. “You cannot be certain about uncertainty”. Uncertainty quantification plays an essential role in decision making, solutions of partial differential equations, optimal design, etc. However, quantifying the uncertainty is not trivial in computational science. The difficulty of uncertainty quantifications increases dramatically with for instance, the dimensionality of the problems, the type of noise in the data, the complexity of black box functions where the data is obtained (large scale simulations, expensive experiments), etc. Moreover, with the knowledge of physics, how to quantify uncertainty with only a few numbers of data should be considered properly.
Uncertainty quantification and its interactions with deep learning is a recently emerging interdisciplinary area that leverages the power of statistical methods, machine learning models, numerical methods and data-driven approach to provide reliable inference for quantities of interest in natural science and engineering problems. Given the fast growth in deep learning, probabilistic methods and the large volume of data available across different research areas (computer visions, natural language processing, decision making), we aim in taking advantage of these recent advances to propose novel methodologies to solve problems where uncertainty quantification plays important roles.
This talk will highlight our recent process on the interaction between deep learning and uncertainty quantifications in the aspects of methodologies and applications. On the methodology side, solutions regarding partial differential equations (forward problems) and system identifications (inverse problems) will be discussed with robust uncertainty estimations. Active learning and data acquisition will also be covered in the presence of uncertainty. Equipped with the methodologies we have developed, we will briefly discuss some applications of such uncertainty quantification techniques in real-world problems such as cardiac flow activation mapping, optimal design as well as analysis for high dimensional data.
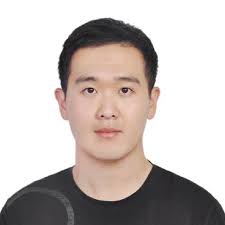
Yibo Yang
Ph.D. Candidate, Department of Mechanical Engineering and Applied Mechanics, University of Pennsylvania
Advisor: Paris Perdikaris