
- This event has passed.
MEAM Seminar: “Computationally-constrained Dynamically-feasible Search-based Motion Planning”
June 20, 2023 at 10:00 AM - 11:30 PM
Planning fast and autonomous robotic motion in unstructured, cluttered environments remains a core challenge of the robotics community. The robot’s motion must be agile and dynamic, operating near the limit of its physical capabilities, to reach its goal location as quickly as possible. Fast motion in turn creates a need for fast plan computation over large and complex areas of the robots environment. Search-based planning, entailing search over trajectory-based graphs, offers a theoretically grounded method to plan optimal motion that is dynamically feasible. However, the computational footprint of this approach is often too burdensome, as graph search speed scales inversely with the size of the planning graph. To tackle this challenge, we concentrate on designing a sparse planning graph and dynamically determining the required graph size based on obstacle density.
We introduce a method for selecting vertices and edges in a motion primitive graph grounded in statistical dispersion, which ensures guarantees on planner completeness. By minimizing dispersion of graph vertices in the trajectory cost-induced metric space, our approach efficiently covers the space of feasible trajectories. Our motion primitive graphs outperform baseline methods, with lower dispersion, fewer iterations of graph search, and fewer tunable parameters.
While this method can generate high-quality graphs of a specified size, the selection of this size has significant effects on planner performance. Sparser graphs may miss a narrow corridor that the plan must traverse, while denser ones may result in excessive computation time. We address this tradeoff with a framework consisting of two parts: offline maximization of planner completeness for several graph sizes, and online dynamic adjustment of the graph size based on empirical planner performance. Through real world experiments in cluttered pine forests, we demonstrate the real-time adaptability of the planner to different environments, enabling flight up to 2.5 m/s in varying tree densities.
Finally, we explore the integration of trajectory optimization with search-based planning, highlighting the potential synergies between these approaches and providing design tradeoffs.
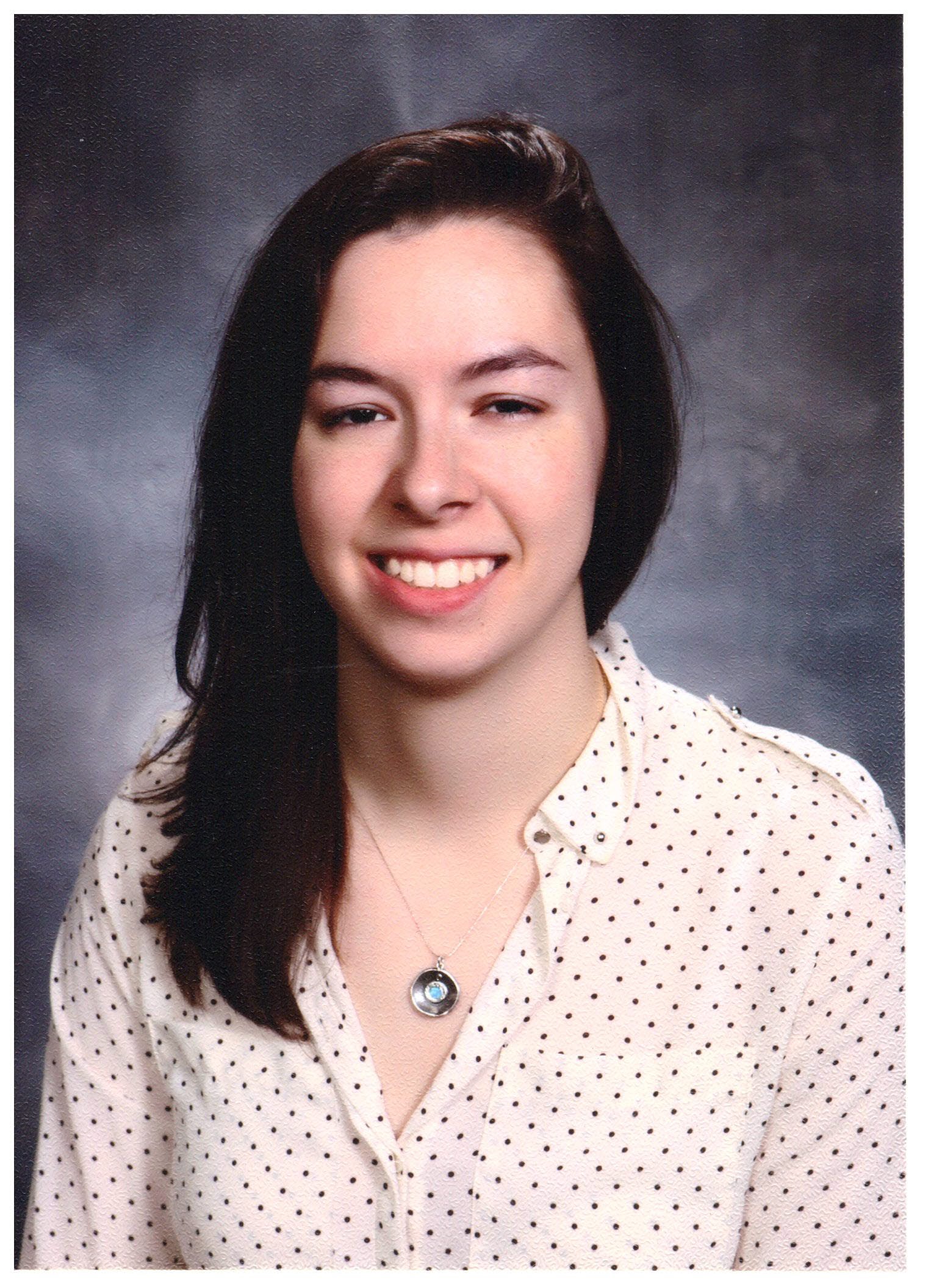
Laura Jarin-Lipschitz
Ph.D. Candidate, Department of Mechanical Engineering & Applied Mechanics, University of Pennsylvania
Advisors: Mark Yim & Vijay Kumar