
- This event has passed.
GRASP on Robotics: “Learning and Influencing Conventions in Interactive Robotics”
November 19, 2021 at 10:30 AM - 11:45 AM
*This will be a HYBRID Event with in-person attendance in Wu & Chen Auditorium and Virtual attendance via Zoom Webinar here.
There have been significant advances in the field of robot learning in the past decade. However, many challenges still remain when studying how robot learning can advance interactive agents such as robots that collaborate with humans, and how interactions can enable more effective robot learning. This introduces an opportunity for developing new robot learning algorithms that can help advance the science of interactive autonomy. In this talk, we will discuss a formalism that learns conventions, i.e., low-dimensional representations sufficient for capturing non-stationary interactions. We demonstrate how we can influence and stabilize these conventions to achieve desirable outcomes in multi-robot coordination. Finally, we will then talk about some of the challenges of learning such representations when interacting with humans, and how we can develop data-efficient techniques that can tap into different sources of data such as suboptimal demonstrations or can actively learn human preferences. We will end the talk with a discussion of applications of these techniques in assistive robotics.
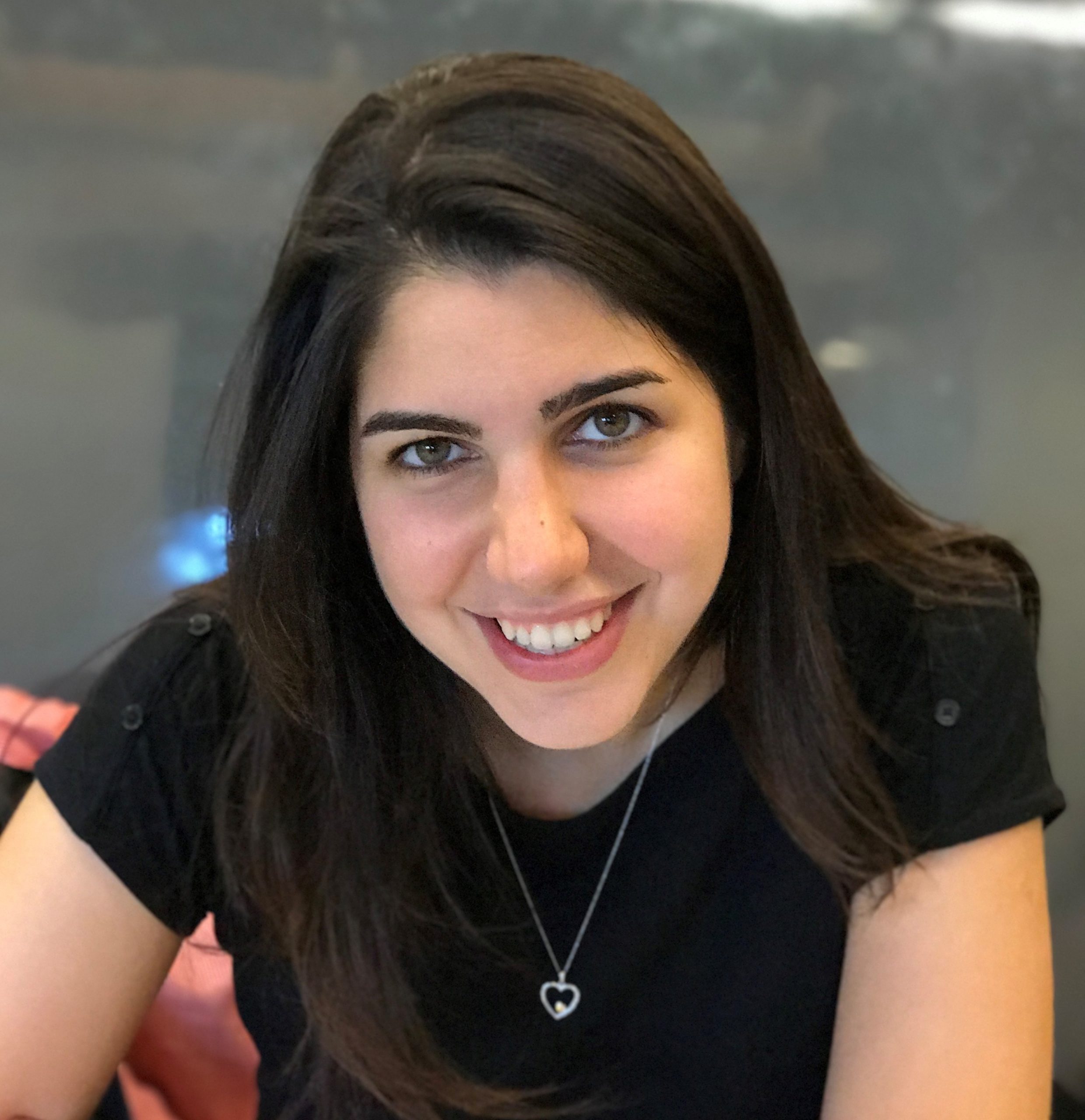
Dorsa Sadigh
Stanford University
Dorsa Sadigh is an assistant professor in Computer Science and Electrical Engineering at Stanford University. Her research interests lie in the intersection of robotics, learning, and control theory. Specifically, she is interested in developing algorithms for safe and adaptive human-robot and multi-agent interaction. Dorsa received her doctoral degree in Electrical Engineering and Computer Sciences (EECS) from UC Berkeley in 2017, and received her bachelor’s degree in EECS from UC Berkeley in 2012. She is recognized by awards such as the NSF CAREER award, the AFOSR Young Investigator award, the IEEE TCCPS early career award, MIT TR35, as well as industry awards such as the JP Morgan, Google, and Amazon faculty research awards.