
- This event has passed.
ESE Thesis Defense: “Constrained Learning and Inference”
September 22, 2020 at 9:00 AM - 10:30 AM
Learning is a core component of the information processing and autonomous systems upon which we increasingly rely on to select job applicants, analyze medical data, and drive cars. As these systems become ubiquitous, so does the need to curtail their behavior. Left untethered, they are susceptible to tampering (adversarial examples) and prone to prejudiced and unsafe actions. Currently, this is done by either constructing models that embed the desired properties or tuning the training objective so as to promote them. Yet, these approaches are often tailored to specific problems, are hard to transfer between models, and involve time consuming trial-and-error procedures that are impractical even for the current scale and complexity of modern machine learning systems. In this defense, I develop the theoretical underpinnings of constrained learning to understand how requirements affect statistical learning and enable behaviors to be directly and systematically designed. To do so, I will derive a generalization theory for constrained learning based on the probably approximately correct (PAC) learning framework. In particular, I will show that imposing requirements does not make a learning problem harder in the sense that any PAC learnable class is also PAC constrained learnable using a constrained counterpart of the empirical risk minimization (ERM) rule. For typical parametrized models, however, this learner involves solving a non-convex constrained optimization program for which even obtaining a feasible solution may be hard. To overcome this issue, we prove that under mild conditions the empirical dual problem of constrained learning is also a PAC constrained learner. Hence, constrained learning problems can be solved by solving only unconstrained ones, leading to a practical constrained learning algorithm. We illustrate how constrained learning can address problems in fair and robust classification.
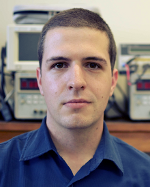