
- This event has passed.
ESE Spring Seminar – “Can Robots Learn from Machine Dreams? – Robot Learning via GenAI-powered World Models”
March 20 at 11:00 AM - 12:00 PM
Over the past decade, large-scale pre-training followed by alignment has revolutionized natural language processing and computer vision. Yet, robotics remains constrained by the scarcity of real-world data. In this talk, I will present our systematic approach to overcoming this bottleneck by building increasingly rich world models from data. I will first introduce our distilled feature field work that represents a robot’s action in relation to multi-modal descriptors in a static environment. Building upon this foundation, I will demonstrate LucidSim, a GenAI-powered simulator that significantly narrows the sim-to-real gap in the visual domain. I will show the initial steps we have taken toward a robot foundation model by extending this framework from legged locomotion to manipulation tasks. These results suggest that synthetic data sourced from world models can accelerate robot learning beyond what is possible with real-world data alone. I will conclude by outlining a roadmap that begins with enabling machines to dream a million worlds that will ultimately allow AI to enter the physical world.
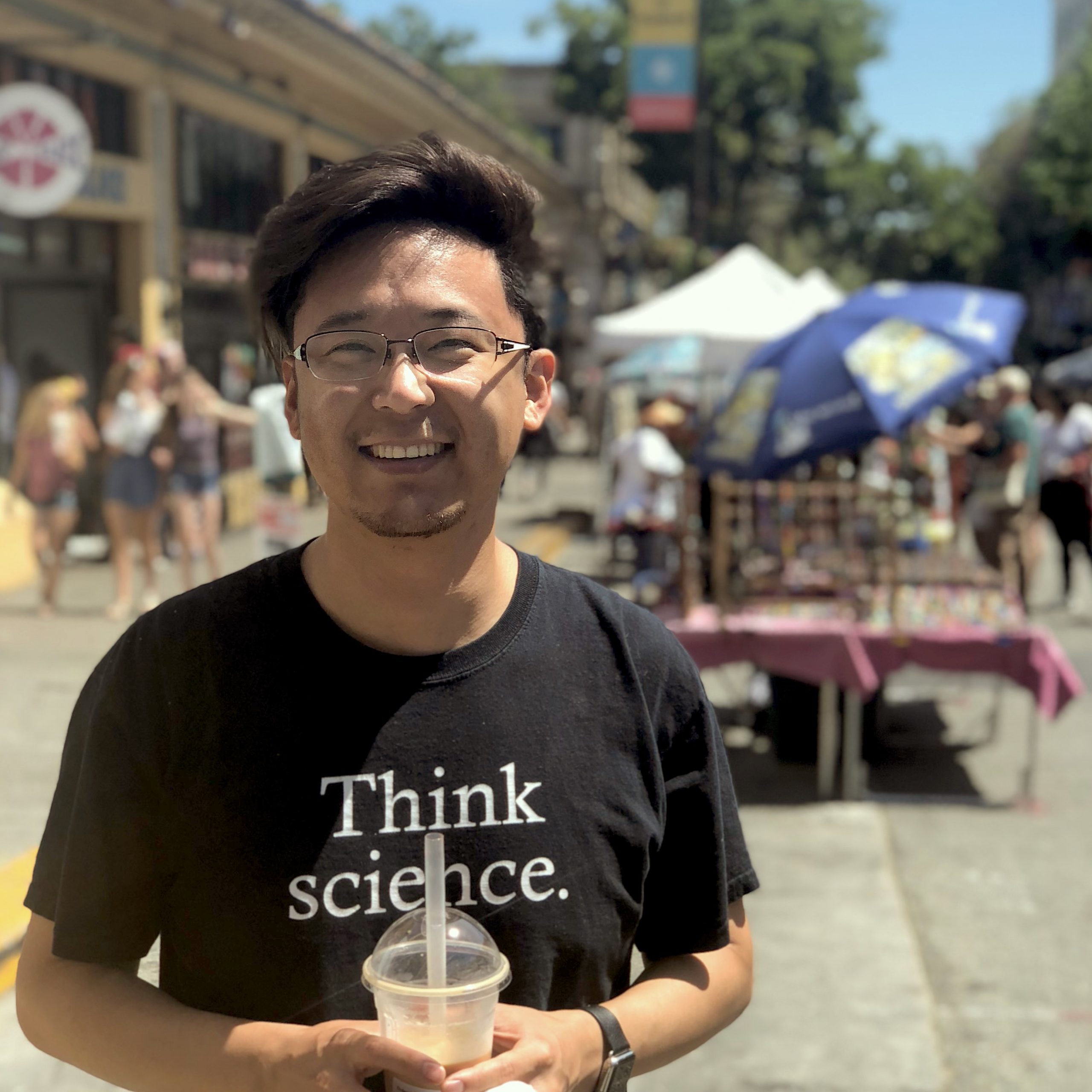
Ge Yang
Postdoctoral Fellow, MIT
Ge Yang is a postdoctoral researcher working with Phillip Isola at MIT CSAIL and collaborate closely with Xiaolong Wang at UCSD. His research focuses on developing algorithmic and system foundations for computational visuomotor learning. Ge is a recipient of the Best Paper Award at the Conference on Robot Learning (CoRL) in 2023 for his work on Distilled Feature Fields, and an NSF postdoc fellowship at the Institute of AI and Fundamental Interactions. Ge’s multi-disciplinary approach in AI was heavily influenced by his prior experience as a physicist. Ge obtained his bachelor’s degree in Physics and Mathematics from Yale University and his Ph.D. from the University of Chicago, advised by David I. Schuster (now at Stanford).