
- This event has passed.
ESE Spring Seminar – “Physics-inspired Machine Learning”
February 12, 2024 at 11:00 AM - 12:00 PM
Combining physics with machine learning is a rapidly growing field of research. Thereby, most work focuses on leveraging machine learning methods to solve problems in physics. Here, however, we focus on the converse, i.e., physics-inspired machine learning, which can be described as incorporating structure from physical systems into machine learning methods to obtain models with better inductive biases. More concretely, we propose several physics-inspired deep learning architectures for sequence modelling based on nonlinear coupled oscillators, Hamiltonian systems and multi-scale dynamical systems. The proposed architectures tackle central problems in the field of recurrent sequence modeling, namely the vanishing and exploding gradients problem as well as the issue of insufficient expressive power. Moreover, we discuss physics-inspired learning on graphs, wherein the dynamics of the message-passing propagation are derived from physical systems. We further prove that these methods mitigate the over-smoothing issue, thereby enabling the construction of deep graph neural networks (GNNs). We extensively test all proposed methods on a variety of versatile synthetic and real-world datasets, ranging from image recognition, speech recognition, natural language processing (NLP), medical applications, and scientific computing for sequence models, to citation networks, computational chemistry applications, and networks of articles and websites for graph learning models. Finally, we show how to leverage physics-based inductive biases of physics-inspired machine learning methods to solve problems in the physical sciences.
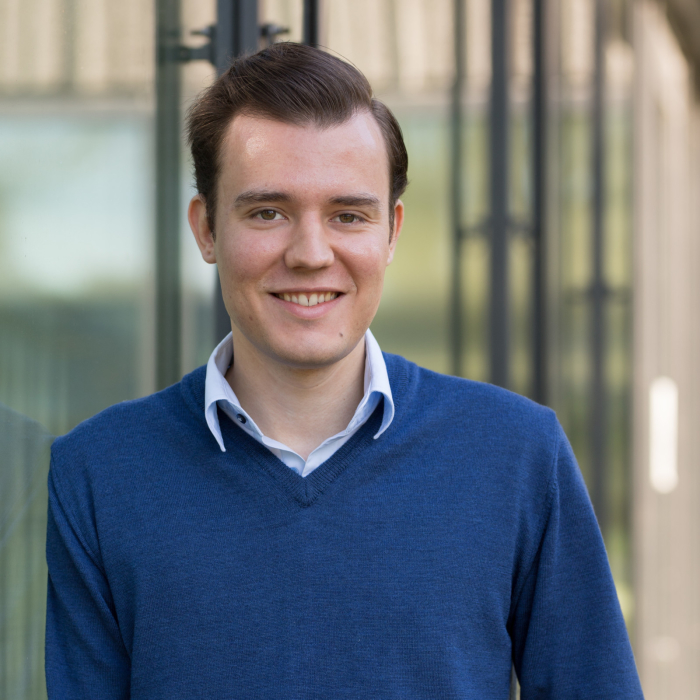
T. Konstantin Rusch
SNSF Postdoctoral Research Fellow, MIT
T. Konstantin Rusch is an SNSF postdoctoral research fellow at CSAIL, MIT. Before that, he obtained a PhD in Applied Mathematics and Machine Learning at ETH Zurich, supervised by Prof. Dr. Siddhartha Mishra. During his doctoral studies, he had a second affiliation at UC Berkeley, advised by Prof. Dr. Michael Mahoney. Moreover, he held visiting research appointments at UC Berkeley and the University of Oxford. His main research interest is in combining physics with machine learning. Thereby, he focuses on physics-inspired machine learning, which can be described as leveraging structure from physical systems to construct novel machine learning methods with better inductive biases. Additionally, he works on combining methods from numerical analysis with machine learning to solve problems in computational science and engineering.