
- This event has passed.
ESE Spring Colloquium – “Towards Fair and Efficient Machine Learning with Large Models”
February 22, 2022 at 11:00 AM - 12:00 PM
Deep networks often achieve better accuracy as we employ larger models. However, modern machine learning applications involve multiple considerations alongside accuracy, such as resource-efficiency, robustness, or fairness. Deploying ML in the real-world requires sound solutions addressing these considerations.
In this talk, I will first discuss optimizing fairness objectives for imbalanced data. We observe that a large model can easily achieve “perfect fairness” on training data but dramatically fail at the test-time due to overfitting. To address this, we propose two strategies, (1) A new family of fairness-seeking loss functions, (2) Algorithms that optimize validation (rather than training) objective, and combine them to achieve state-of-the-art performance. We also introduce new optimization methods that extend these to decentralized settings.
I will then discuss training efficient sparse models. While conventional wisdom strongly advocates the use of regularization, we observe that perfectly fitting a large model to data and then pruning it achieves stellar accuracy. We demystify this surprising feature-selection ability through a flexible theory which can answer “How good is the pruned model?”.
In summary, our results provide several insights on learning with large models: (1) Our theory based on linear and random-feature models provide useful intuitions for understanding modern deep learning, (2) Large models can benefit from unconventional training strategies such as new loss functions, and (3) Validation phase is particularly helpful for large models that are susceptible to overfitting.
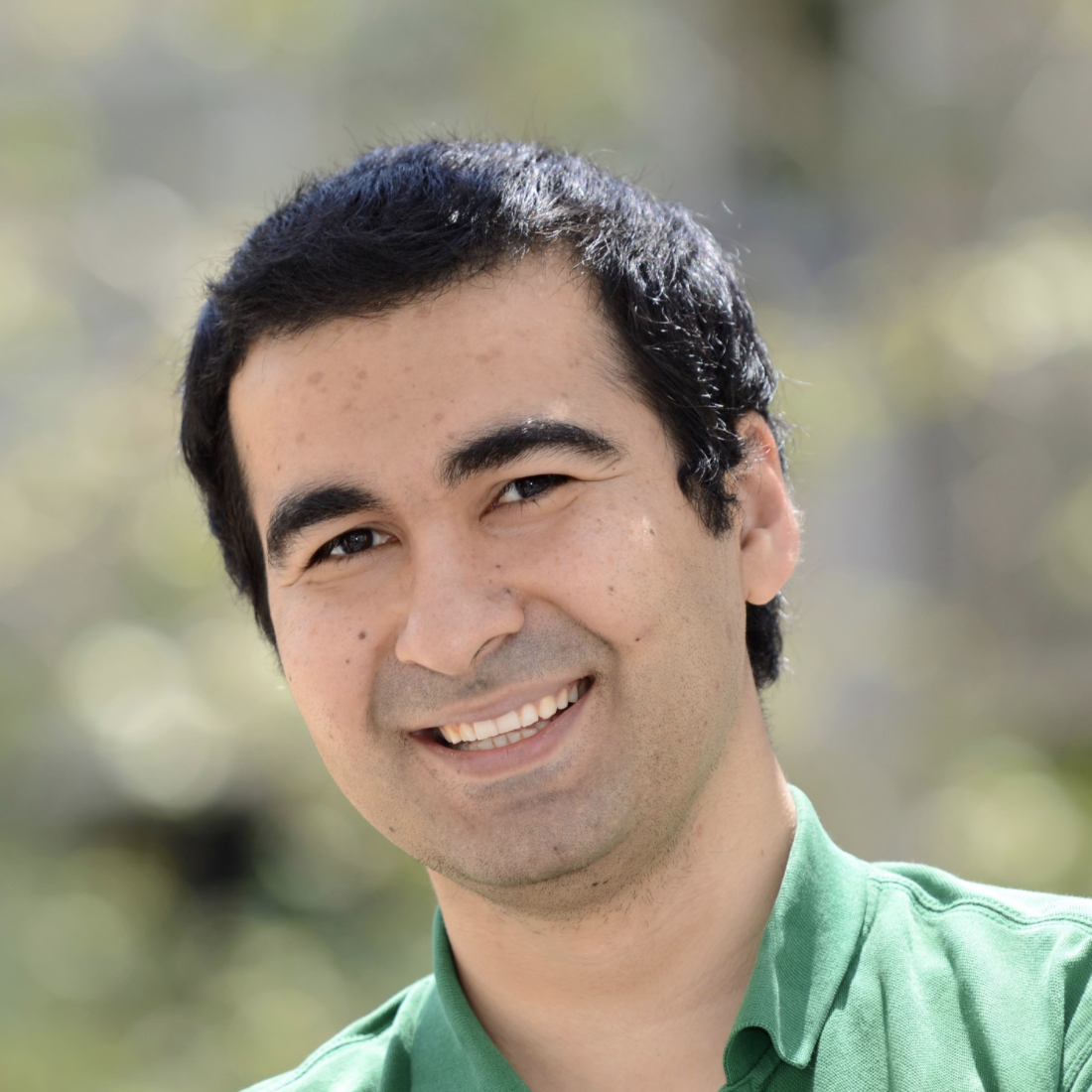
Samet Oymak
Assistant Professor of Electrical and Computer Engineering, UC Riverside
Samet Oymak is an assistant professor of Electrical and Computer Engineering at the University of California, Riverside since 2018. Prior to UCR, he spent three years at Google and in algorithmic finance. During his postdoc, he was at UC Berkeley as a Simons Fellow and a member of AMPLab. He obtained his bachelor’s degree from Bilkent University in 2009 and his PhD degree from Caltech in 2015. He received the Charles Wilts Prize for the best departmental PhD thesis at Caltech in 2015 and the NSF CAREER award in 2021. Website: sametoymak.com