
- This event has passed.
ESE PhD Thesis Defense: “Scalable and Risk-Aware Verification of Learning Enabled Autonomous Systems”
April 10 at 1:30 PM
As autonomous systems become more prevalent, ensuring their safety will become more and more important. However, deriving guarantees for these systems is becoming increasingly difficult due to the use of black box, learning enabled components and the growing range of operating domains in which they are deployed. The complexity of the learning-enabled components greatly increases the computational complexity of the verification problem. Additionally, the safety predictions from verifying these systems must be conservative. This thesis explores two high-level methods for verifying autonomous systems: probabilistic model checking and statistical model checking. Probabilistic model checking methods exhaustively analyze a model of the system to reason about its properties. These methods generally suffer from scalability issues, but if the abstraction is built correctly then the results will be provably conservative. On the other hand, statistical model checking methods draw traces from the system to reason about its properties. These methods don’t suffer the scalability drawback of probabilistic model checking, but their guarantees are weaker and may not even be conservative. This thesis introduces methods for improving the scalability of verifying autonomous systems with probabilistic model checking methods and incorporating notions of conservatism into statistical model checking.
On the probabilistic model checking side, this thesis first explores using engineering intuitions about systems to reduce probabilistic model checking complexity while preserving conservatism. Next, standard conservative probabilistic model checking techniques are used to synthesize runtime monitors that are conservative and lightweight. Finally, this thesis presents a run-time method for composing monitors of verification assumptions. Verification assumptions are critical for simplifying verification problems so that they become computationally feasible.
For statistical model checking, this thesis first leverages a method called conformal prediction to bound the errors of trajectory predictors, which enables safe (i.e. conservative) planning in dynamic environments. Additionally, a method for producing less conservative conformal prediction regions in time series settings is developed. Then a method called risk verification is developed, which uses statistical methods to bound risk metrics of a system’s performance. Risk metrics, which capture tail events of the system’s performance, offer a statistical equivalent of worst case analysis.
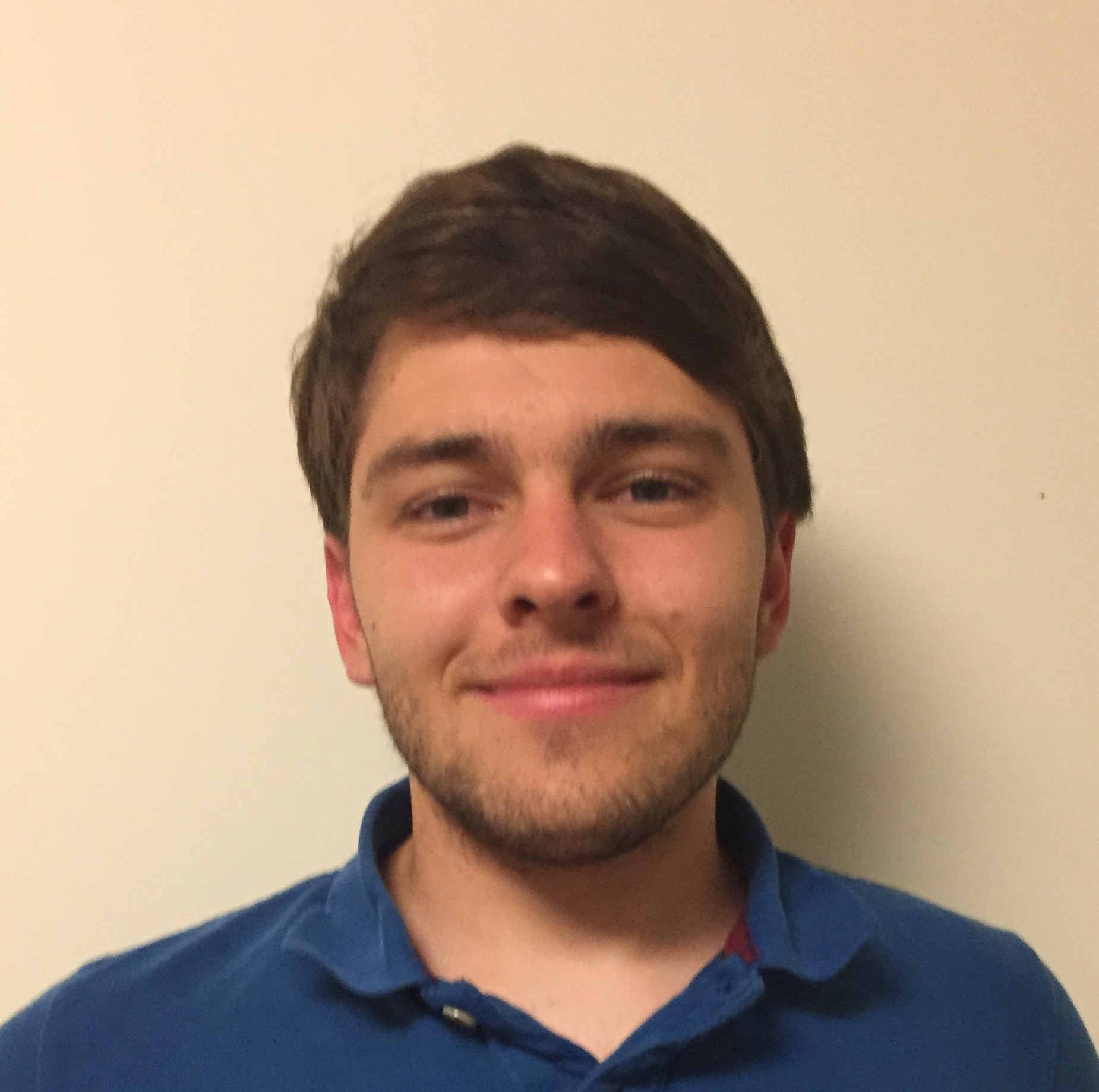
Matthew Cleaveland
Ph.D. Candidate
Matthew Cleaveland is a PhD candidate at the University of Pennsylvania in the Electrical and Systems Engineering department. His research focuses on reasoning about safety properties of autonomous and cyber-physical systems. He completed his undergraduate education at Duke University in 2018, where he majored in math and electrical and computer engineering.