
- This event has passed.
ESE PhD Thesis Defense: “Learning, Privacy, and Reliable Communication in Large Data Networks”
November 22, 2023 at 10:00 AM - 12:00 PM
This thesis explores advancements in three distinct domains: communications, privacy, and machine learning. Within the realm of communication, a comprehensive study is conducted on channel coding at low capacity, a critical aspect of Internet of Things (IoT) technology requiring reliable transmission over channels with minimal capacity. Despite existing finite-length analyses yielding inaccurate predictions and current coding schemes proving inefficient in low-capacity scenarios, this thesis addresses these limitations. It characterizes the finite-length fundamental limits of channel coding for essential channels, offering provably efficient code designs tailored for low-capacity environments. In the domain of privacy, attention is directed towards a decentralized consensus problem handled in a private manner. Existing methods for classical consensus problems often involve openly exchanging private information. This thesis proposes an algorithmic framework capable of achieving the exact limit and the fastest possible convergence rate while safeguarding the privacy of users’ local values. Additionally, a novel information-theoretic metric is introduced to effectively measure the privacy of a node concerning another node within the network. In the machine learning domain, a rigorous mathematical framework is established to investigate the function class of Graph Neural Networks (GNNs) in relation to their initialization and internal estimators. Utilizing a unique technique for the algebraic representation of a multiset of vectors, termed Multiset Equivalent Function (MEF), it is demonstrated that GNNs can generate functions satisfying a weight-equipped variant of permutation-equivariance using distinct features. The MEF technique further reveals that GNNs, equipped with node identifiers in their initialization, can generate any function in a fully connected and weighted graph scenario. These findings contribute to a formal understanding of the intricate relationship between GNNs and other algorithmic procedures applied to graphs, such as min-cut value and shortest path problems, as well as the re-derivation of the known connection between GNNs and the Weisfeiler-Lehman graph-isomorphism test.
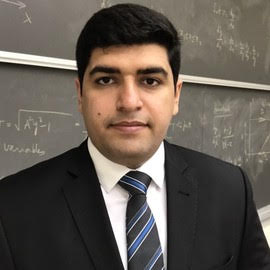
Mohammad Fereydounian
ESE Ph.D. Candidate
Mohammad Fereydounian received two B.Sc. degrees in pure mathematics and in electrical engineering and the M.Sc. degree in pure mathematics from the Sharif University of Technology, Tehran, Iran, in 2014 and 2016, respectively, and the A.M. degree in statistics from the Wharton School, University of Pennsylvania, in 2021, where he is currently a Ph.D. candidate in Electrical and Systems Engineering Department. His research interests include information and coding theory, optimization, and machine learning. He was awarded ESE Best Teaching Assistant Award for A Doctoral Student in 2018. He also nominated for Graduate Fellowship for Teaching Excellence from University of Pennsylvania’s Center for Teaching and Learning (CTL) in 2020. He also received Certificate in College and University Teaching from CTL in 2019.