
- This event has passed.
ESE PhD Thesis Defense: “Discrete and Continuous Optimization for Collaborative and Multi-task Learning”
July 14, 2023 at 11:00 AM - 1:00 PM
This thesis is dedicated to addressing the challenges of robust collaborative learning and optimization in both discrete and continuous domains. With the ever-increasing scale of data and the growing demand for effective distributed learning, a multitude of obstacles emerge, including communication limitations, resilience to failures and corrupted data, limited information access, and collaboration in multi-task learning scenarios. The thesis consists of seven chapters, each targeting specific aspects of these challenges.
In the first chapter, novel algorithms are introduced for collaborative linear bandits, offering a comprehensive exploration of the benefits of collaboration in the presence of adversaries through thorough analyses and lower bounds. The second chapter delves into multi-agent min-max learning problems by tackling the presence of Byzantine adversarial agents. Chapter three delves into the effects of delays within stochastic approximation schemes, investigating non-asymptotic convergence rates under Markovian noise.
Moving forward, the fourth chapter focuses on analyzing the performance of standard min-max optimization algorithms with delayed updates. The fifth chapter concentrates on robustness in discrete learning, specifically addressing convex-submodular problems in mixed continuous-discrete domains. The sixth chapter tackles the challenge of limited information access in collaborative problems with distributed constraints, developing optimal algorithms for submodular maximization under distributed partition matroid constraints.
Lastly, the seventh chapter introduces a discrete variant of multi-task learning and meta-learning. In summary, this thesis contributes to the field of robust collaborative learning and decision-making by providing insights, algorithms, and theoretical guarantees in discrete and continuous optimization. The advancements made across linear bandits, minimax optimization, distributed robust learning, delayed optimization, and submodular maximization pave the way for future developments in collaborative and multi-task learning.
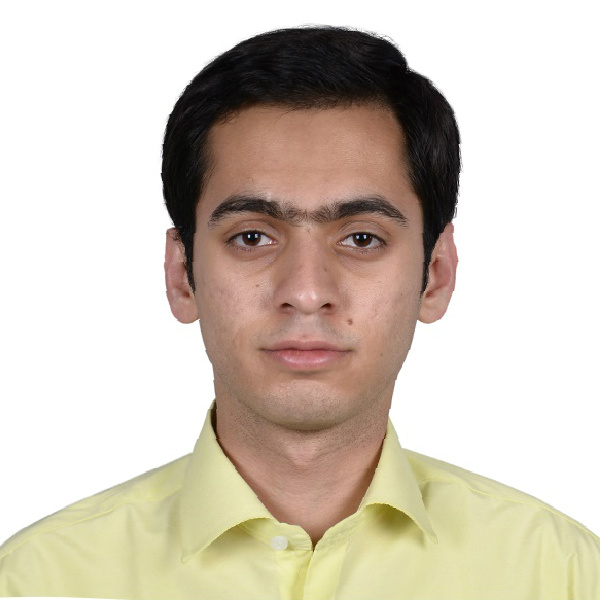
Arman Adibi
ESE Ph.D. Candidate
Arman Adibi graduated from Isfahan University of Technology in 2018 with a BSc in Electrical Engineering and a minor in Mathematics. Currently, he is pursuing a Ph.D. in electrical and systems engineering at the University of Pennsylvania. His research focuses on submodular optimization, distributed learning, robust learning, and reinforcement learning.