
- This event has passed.
ESE PhD Thesis Defense: “Algorithms for Adversarially Robust Deep Learning”
June 4 at 1:00 PM - 3:00 PM
Given the widespread use of deep learning models in safety-critical applications, ensuring that the decisions of such models are robust against adversarial exploitation is of fundamental importance. In this thesis, we discuss recent progress toward designing algorithms that exhibit desirable robustness properties. First, we discuss the problem of adversarial examples in computer vision, for which we introduce new technical results, training paradigms, and certification algorithms. Next, we consider the problem of domain generalization, wherein the task is to train neural networks to generalize from a family of training distributions to unseen test distributions. We present new algorithms that achieve state-of-the-art generalization in medical imaging, molecular identification, and image classification. Finally, we study the setting of jailbreaking large language models (LLMs), wherein an adversarial user attempts to design prompts that elicit objectionable content from an LLM. We propose new attacks and defenses, which represent the frontier of progress toward designing robust language-based agents.
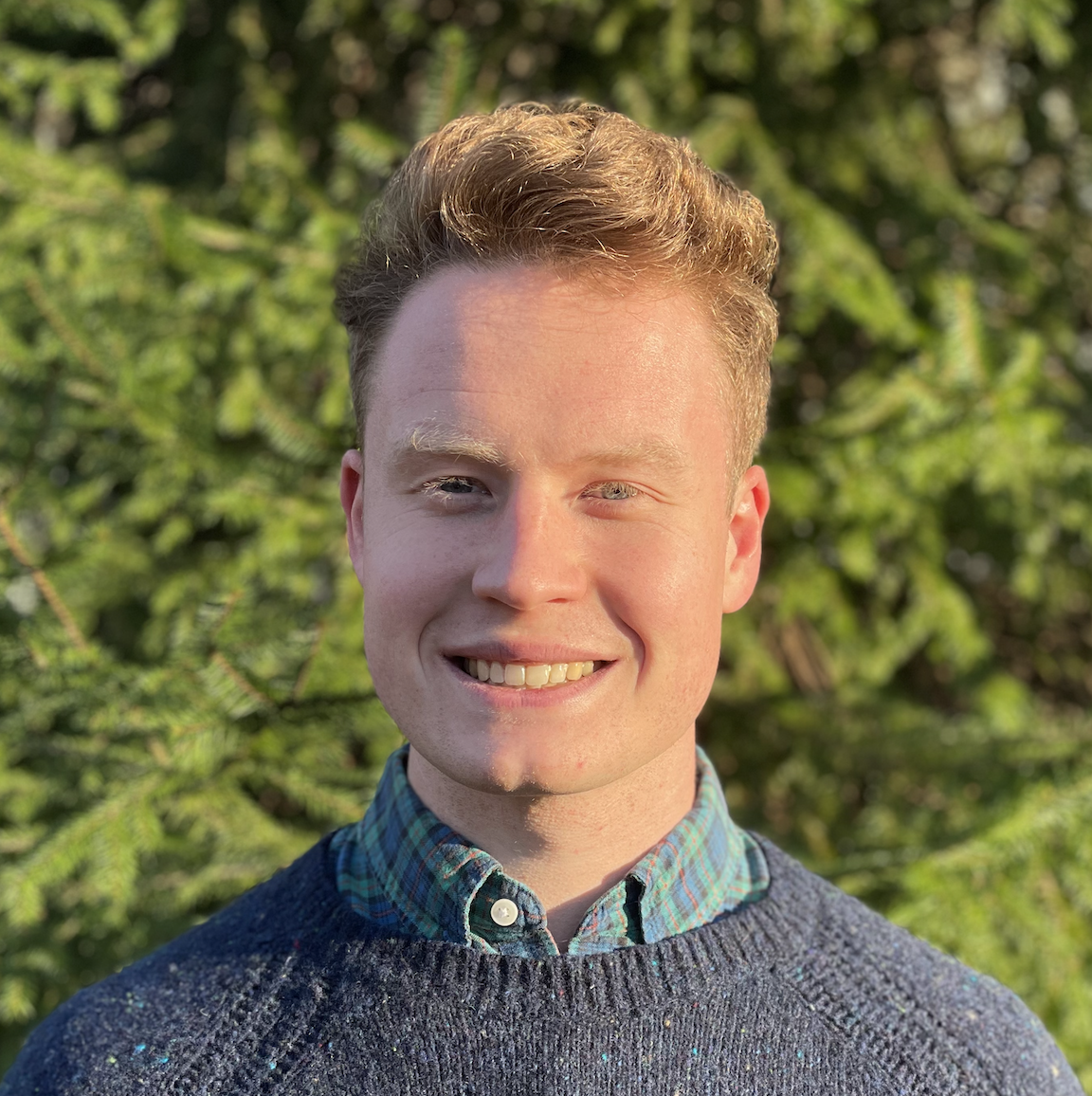
Alex Robey
ESE Ph.D. Candidate
Alex Robey is a Ph.D. candidate in the Department of Electrical and Systems Engineering at the University of Pennsylvania, where he is a member of the ASSET Center for Safe, Explainable, and Trustworthy Machine Learning, the Warren Center for Network and Data Sciences, and the GRASP Robotics Laboratory. He received a B.S. in Engineering and a B.A. in Mathematics from Swarthmore College in 2018. His research addresses real-world robustness challenges in machine learning, with relevant work spanning perturbation-based attacks in computer vision, complex distribution shifts in multimodal data, and AI safety challenges involving large language models.