
- This event has passed.
ESE Ph.D. Thesis Defense: “Training Adaptive and Sample-Efficient Autonomous Agents”
April 16 at 2:30 PM
AI agents, both in the physical and digital worlds, should generalize from their training data to three increasingly difficult levels of deployment: training tasks and environments, training tasks and environments with variations, and completely new tasks and environments. Moreover, like humans, they are expected to learn from as little training data as possible, especially in the physical world, and adapt with as little adaptation data as possible. This thesis is founded around and describes work that tackles these levels of generalization with an additional emphasis on sample-efficiency.
We start with a focus on training data efficiency and the simplest level of generalization from training data to training tasks and environments (a.k.a., level 1). AI agents, especially in the physical world, are usually trained via one of two paradigms: imitation learning or reinforcement learning. First, we propose a plug-in model class to improve behavior cloning with any deep neural network (DNN) backbone that is particularly effective in the low-data regime. Second, we leverage our proposed model class to guarantee the conformance of any DNN world model to physics and medical constraints, in a highly data-efficient manner. Third, we improve the sample-efficiency of reinforcement learning agents, by an order of magnitude, by leveraging expert interventions.
Next, we tackle the challenge of generalization to training tasks and environments with variations as well as completely new tasks and environments (a.k.a., levels 2 and 3), keeping both training and adaptation sample-efficiency in mind. Here, we pre-train REGENT, a retrieval-augmented generalist agent that can adapt to unseen robotics and game-playing environments via in-context learning, without any finetuning. REGENT outperforms state-of-the-art generalist agents after pre-training on an order-of-magnitude fewer datapoints and with up to 3x fewer parameters. We also propose a strategy, inspired by adaptive control, to improve the robustness of the image encoder of REGENT, an essential component for handling environment variations.
Finally, we bring REGENT to the real world by converting a Vision Language Action model (VLA) to a REGENTic VLA capable of generalizing to unseen objects and tasks through retrieval-augmentation and in-context learning. Further task-specific REGENTic-tuning substantially improves reliability, surpassing a VLA directly fine-tuned on the same data.
We conclude by outlining future directions to expand the envelope of tasks and environments to which a general AI agent can adapt.
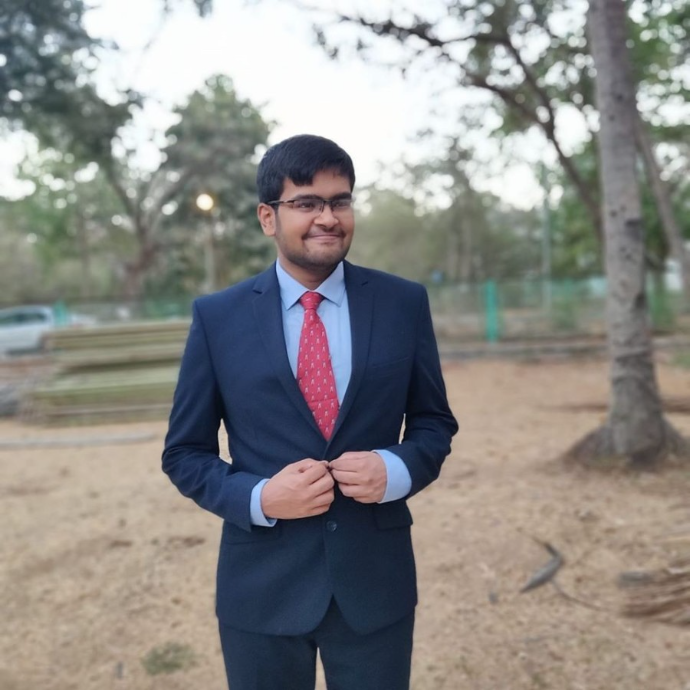
Kaustubh Sridhar
ESE Ph.D. Candidate
Kaustubh is a final-year PhD student at the University of Pennsylvania advised by Insup Lee and closely collaborating with Dinesh Jayaraman. His research focuses on building autonomous agents for the physical and digital worlds. In the past, he has been recognized as an outstanding reviewer in NeurIPS and ICML, and his work was nominated for a best paper award at ICCPS. Kaustubh has interned twice at AWS AI Labs and once at Ford and VW’s self-driving unit. Before starting his PhD, he graduated with honors from the Indian Institute of Technology Bombay.