
- This event has passed.
ESE Ph.D. Thesis Defense: “Statistical Learning for System Identification, Prediction, and Control”
January 20, 2022 at 10:00 AM - 12:00 PM
Despite the recent widespread success of machine learning, we still do not fully understand its fundamental limitations. Going forward, it is crucial to better understand learning complexity, especially in critical decision making applications, where a wrong decision can lead to catastrophic consequences. In this thesis, we focus on the statistical complexity of learning unknown linear dynamical systems, with focus on the tasks of system identification, prediction, and control. We are interested in sample complexity, i.e. the minimum number of samples required to achieve satisfactory learning performance. Our goal is to provide finite-sample learning guarantees, explicitly highlighting how the learning objective depends on the number of samples. A fundamental question we are trying to answer is how system theoretic properties of the underlying process can affect sample complexity.
Using recent advances in statistical learning, high-dimensional statistics, and mini-max theory, we provide finite-sample guarantees in the following settings. i) System Identification. We provide the first finite-sample guarantees for identifying a stochastic partially-observed system; this problem is also known as the stochastic system identification problem. ii) Prediction. We provide the first end-to-end guarantees for learning the Kalman Filter, i.e. for learning to predict, in an offline learning architecture. We also provide the first logarithmic regret guarantees for the problem of learning the Kalman Filter in an online learning architecture, where the data are revealed sequentially. iii) Difficulty of System Identification and Control. Focusing on fully-observed systems, we investigate when learning linear systems is statistically easy or hard, in the finite sample regime. Statistically easy to learn linear system classes have sample complexity that is polynomial with the system dimension. Statistically hard to learn linear system classes have worst-case sample complexity that is at least exponential with the system dimension. We show that there actually exist classes of linear systems which are hard to learn. Such classes include indirectly excited systems with a large degree of indirect excitation. Similar conclusions hold for both the problem of system identification and the problem of learning to control.
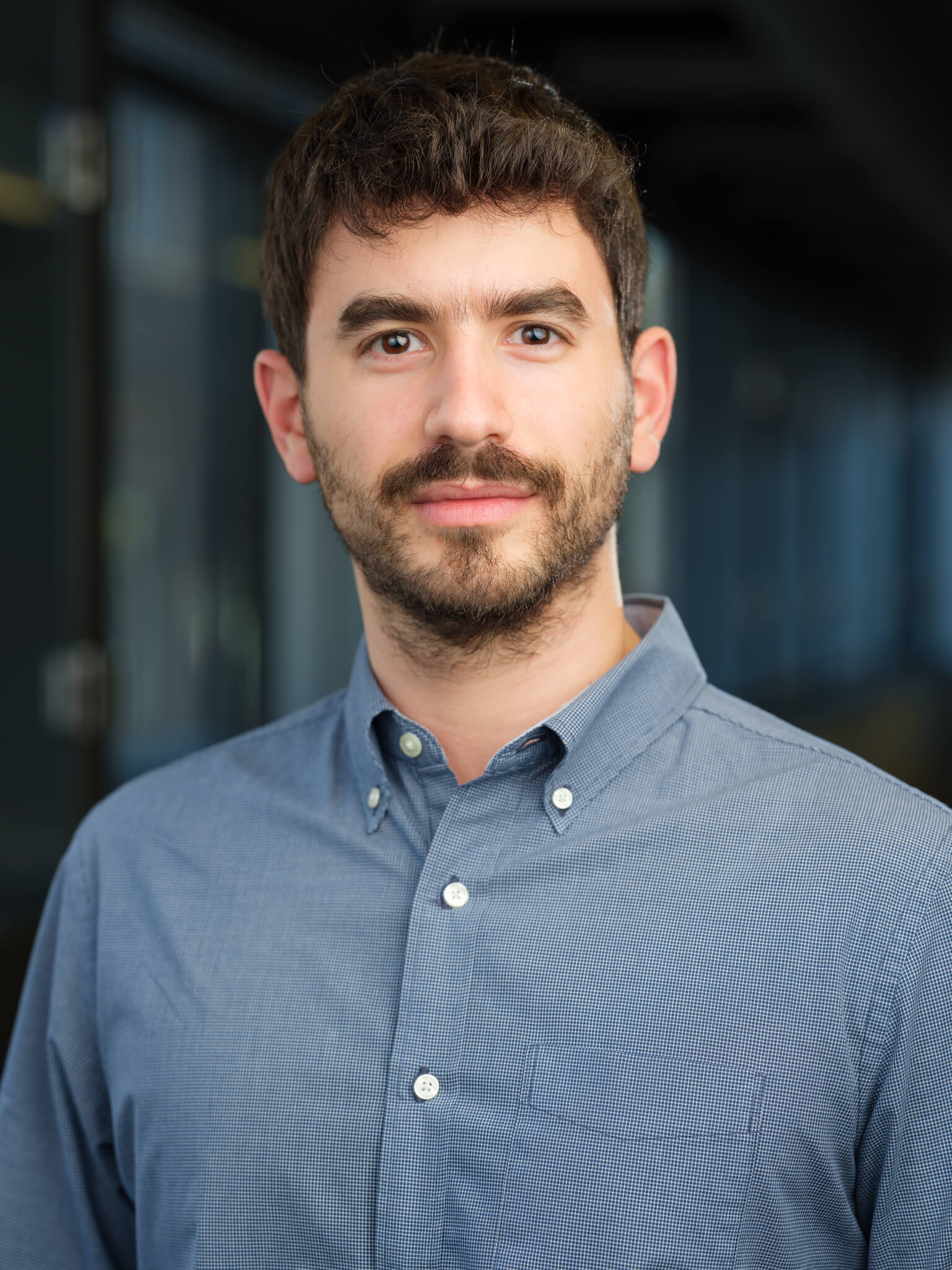
Anastasios Tsiamis
ESE Ph.D. Candidate
Anastasios Tsiamis received the Diploma degree in electrical and computer engineering from the National Technical University of Athens, Greece, in 2014. Currently, he is a Ph.D. student in the Department of Electrical and Systems Engineering at the University of Pennsylvania, advised by George J. Pappas. His research interests include statistical learning for control, risk-aware control and optimization, and networked control systems.
Anastasios Tsiamis was a finalist for the IFAC Young Author Prize in IFAC 2017 World Congress and a finalist for the Best Student Paper Award in ACC 2019.