
- This event has passed.
ESE Ph.D. Thesis Defense: “Machine Learning on Large-Scale Graphs”
June 27, 2022 at 10:00 AM - 12:00 PM
Graph neural networks (GNNs) are successful at learning representations from most types of network data but suffer from limitations in large graphs, which do not have the Euclidean structure that time and image signals have in the limit. Yet, large graphs can often be identified as being similar to each other in the sense that they share structural properties. Indeed, graphs can be grouped in families converging to a common graph limit — the graphon. A graphon is a bounded symmetric kernel which can be interpreted as both a random graph model and a limit object of a convergent sequence of graphs. Graphs sampled from a graphon almost surely share structural properties in the limit, which implies that graphons describe families of similar graphs. We can thus expect that processing data supported on graphs associated with the same graphon should yield similar results. In my research, I formalize this intuition by showing that the error made when transferring a GNN across two graphs in a graphon family is small when the graphs are sufficiently large. This enables large-scale graph machine learning by transference: training GNNs on moderate-scale graphs and executing them on large-scale graphs.
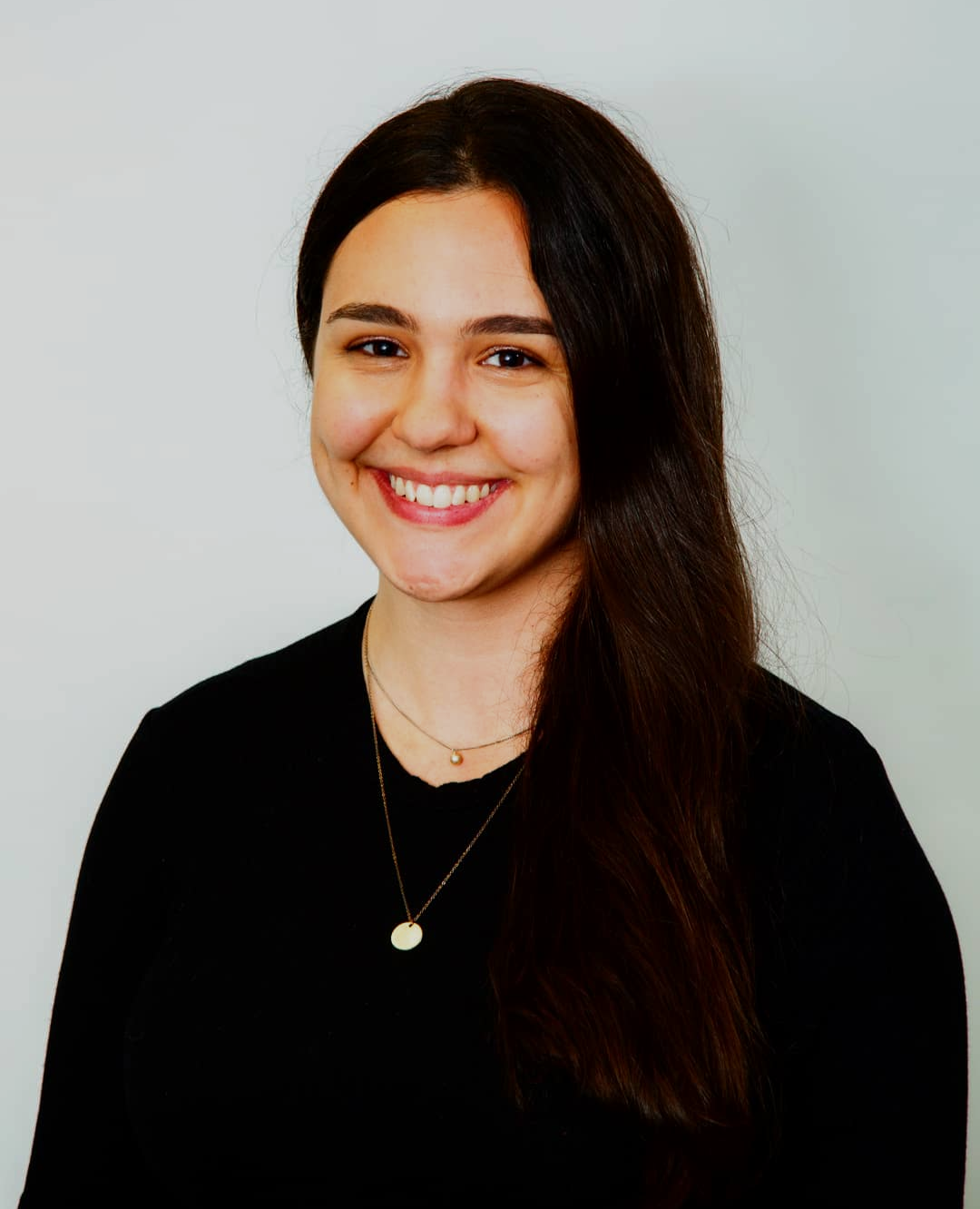
Luana Ruiz
ESE Ph.D. Candidate
Luana Ruiz received the B.Sc. degree in electrical engineering from the University of São Paulo, Brazil, and the M.Eng. degree in electrical engineering from the École Supérieure d’Electricité (now CentraleSupélec), France, in 2017. She is currently a Ph.D. candidate with the Department of Electrical and Systems Engineering at the University of Pennsylvania. Her primary research interests are in large-scale graph machine learning and the mathematical foundations of deep learning. Luana was awarded an Eiffel Excellence scholarship from the French Ministry for Europe and Foreign Affairs between 2013 and 2015, and nominated an iREDEFINE fellow in 2019 and a MIT EECS Rising Star in 2021. She has also received two Best Student Paper awards at the European Signal Processing Conference (EUSIPCO), in 2019 and 2021.