
- This event has passed.
CIS Seminar: “Trustworthy Machine Learning Systems via PAC Uncertainty Quantification”
February 1, 2022 at 3:30 PM - 4:30 PM
Machine learning models are increasingly being incorporated into real-world systems, targeting domains such as robotics, healthcare, and software systems. A key challenge is ensuring that such systems are trustworthy. I will describe a novel strategy for composing machine learning models while providing provable correctness guarantees. First, we show how to quantify the uncertainty of any given model in a way that satisfies PAC correctness guarantees. Second, we show how to compose guarantees for individual models to obtain a guarantee for the overall system. Then, I will discuss applications to ensuring safety in reinforcement learning from visual inputs, and to speeding up inference time of deep neural networks. I will conclude with ongoing work on preserving correctness guarantees in the face of distribution shift.
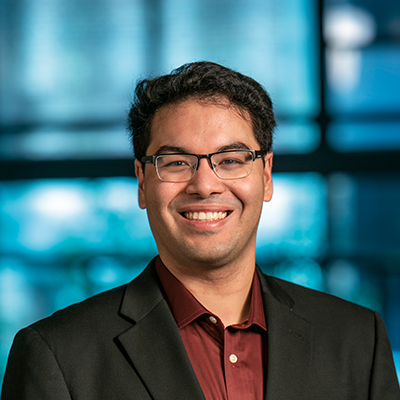
Osbert Bastani
Department of Computer and Information Science, University of Pennsylvania
Osbert Bastani is a research assistant professor at the Department of Computer and Information Science at the University of Pennsylvania. He is broadly interested in techniques for designing trustworthy machine learning systems, focusing on their correctness, programmability, and efficiency. Previously, he completed his Ph.D. in computer science from Stanford and his A.B. in mathematics from Harvard.