
- This event has passed.
CIS Seminar: “Trustworthy Forecasting Algorithms”
September 12, 2024 at 3:30 PM - 4:30 PM
Algorithms are increasingly tasked with forecasting the probabilities of uncertain events: a creditor repaying a loan, a user clicking an advertisement, or a word appearing next in a stream of text, for example. Such forecasts are trustworthy if their users can be sure they won’t regret treating the predicted probabilities as if they were actual distributions from which outcomes would be sampled. The term “calibration” refers to various measures of forecast accuracy that formalize this property of trustworthiness. Defining calibration, and designing algorithms to achieve it, turns out to be a tightrope walk between strong definitions, which ensure reliable results for users but are computationally and statistically harder to achieve, and weak definitions, which have the opposite benefits and drawbacks. I will report on some recent research aimed at locating a sweet spot between these two extremes: calibration properties that are achievable using no more samples or computation than the easiest statistical learning tasks, while providing guarantees for downstream users that are, in many cases, as powerful as the strongest notions of calibration.
This talk is based on joint work with Michael Kim, Princewill Okoroafor, Renato Paes Leme, Jon Schneider, and Yifeng Teng.
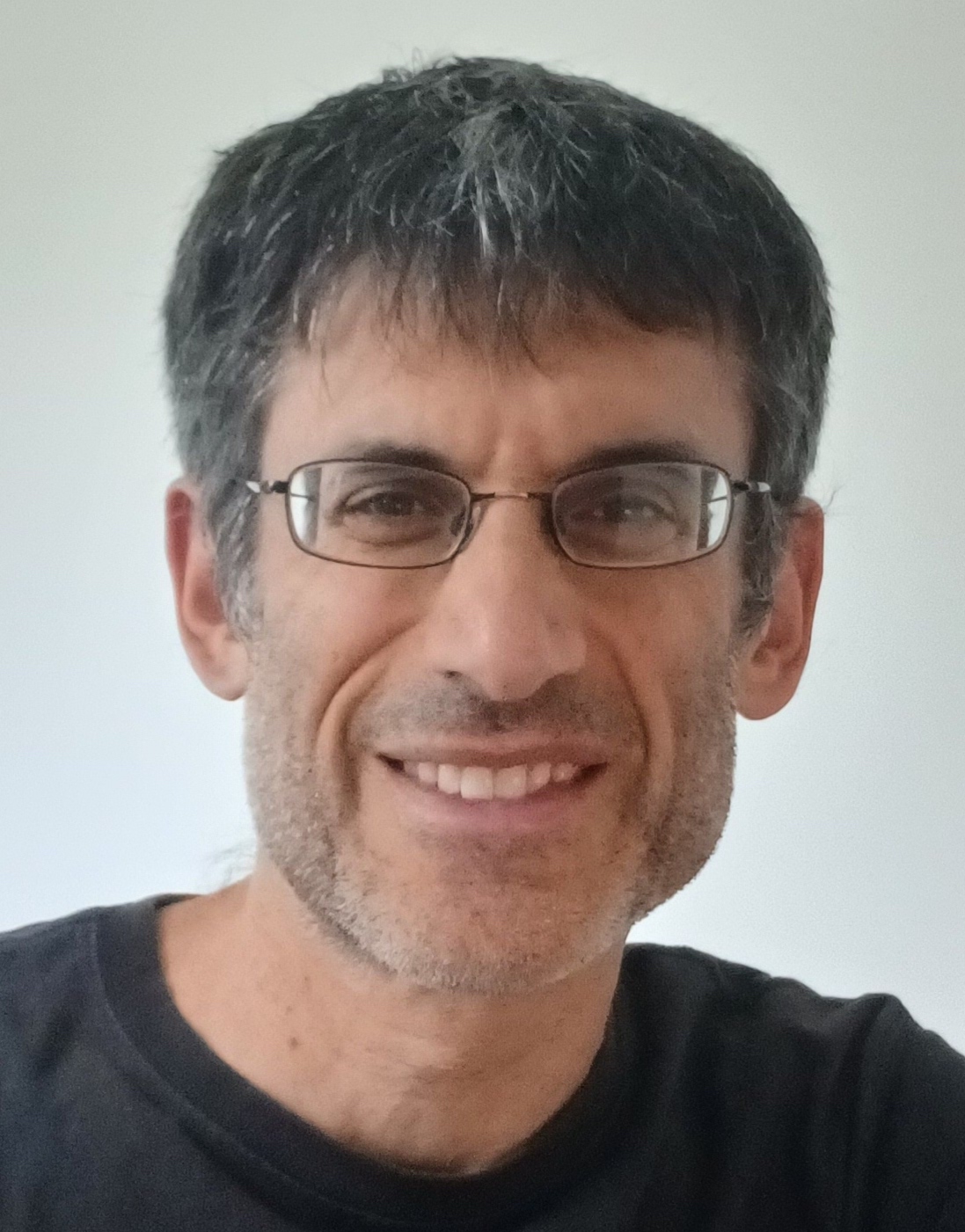
Robert Kleinberg
Professor of Computer Science, Cornell University
Bobby Kleinberg is a Professor of Computer Science at Cornell University and a part-time Faculty Researcher at Google. His research concerns algorithms and their applications to machine learning, economics, networking, and other areas. Prior to receiving his doctorate from MIT in 2005, Kleinberg spent three years at Akamai Technologies; he and his co-workers received the 2018 SIGCOMM Networking Systems Award for pioneering the first Internet content delivery network. He is a Fellow of the ACM and a recipient of the ACM SIGecom Mid-Career Award for advancing the understanding of on-line learning and decision problems and their application to mechanism design.