
- This event has passed.
CIS Seminar: “Principled Algorithm Design in the Era of Deep Learning”
February 16, 2022 at 3:30 PM - 4:30 PM
Deep learning has seen tremendous growth in the last decade with applications across almost all fields of science and technology. In the pursuit of making deep learning methods more efficient and adaptable, there is an increasing need to design better algorithms and architectures. In this talk, I will give an overview of my research efforts towards advancing the statistical and computational foundations of deep learning with the goal of designing new principled algorithms and models. I will show how techniques originally developed for classical learning theory and convex optimization can be combined and extended for the era of deep learning. I will highlight this through two main contributions:
(1) New algorithms for training basic deep learning architectures that are simple, computationally efficient, and provably succeed even when the standard pipelines fail,
(2) A statistical characterization of state-of-the-art attention architectures, like Transformers, that gives new insights on their ability to capture long-range dependencies.
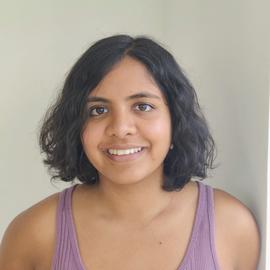
Surbhi Goel
Postdoctoral Researcher, Microsoft Research NYC
Surbhi Goel is currently a postdoctoral researcher at Microsoft Research NYC with the Machine Learning group. She received her Ph.D. from the Department of Computer Science at the University of Texas at Austin where she was advised by Adam Klivans. Her work lies at the intersection of machine learning and theoretical computer science, with a focus on developing the statistical and computational foundations of modern machine learning paradigms, especially deep learning. Among other honors, she is a recipient of UT Austin’s Bert Kay Dissertation award, a J.P. Morgan AI PhD fellowship, a Simons-Berkeley research fellowship, and several fellowships from UT Austin. She has also been recognized as a Rising Star in ML by University of Maryland and in EECS by UIUC.