
- This event has passed.
CIS Seminar: “Learning with Label Noise: A Progressive Approach”
April 21, 2021 at 3:00 PM - 4:00 PM
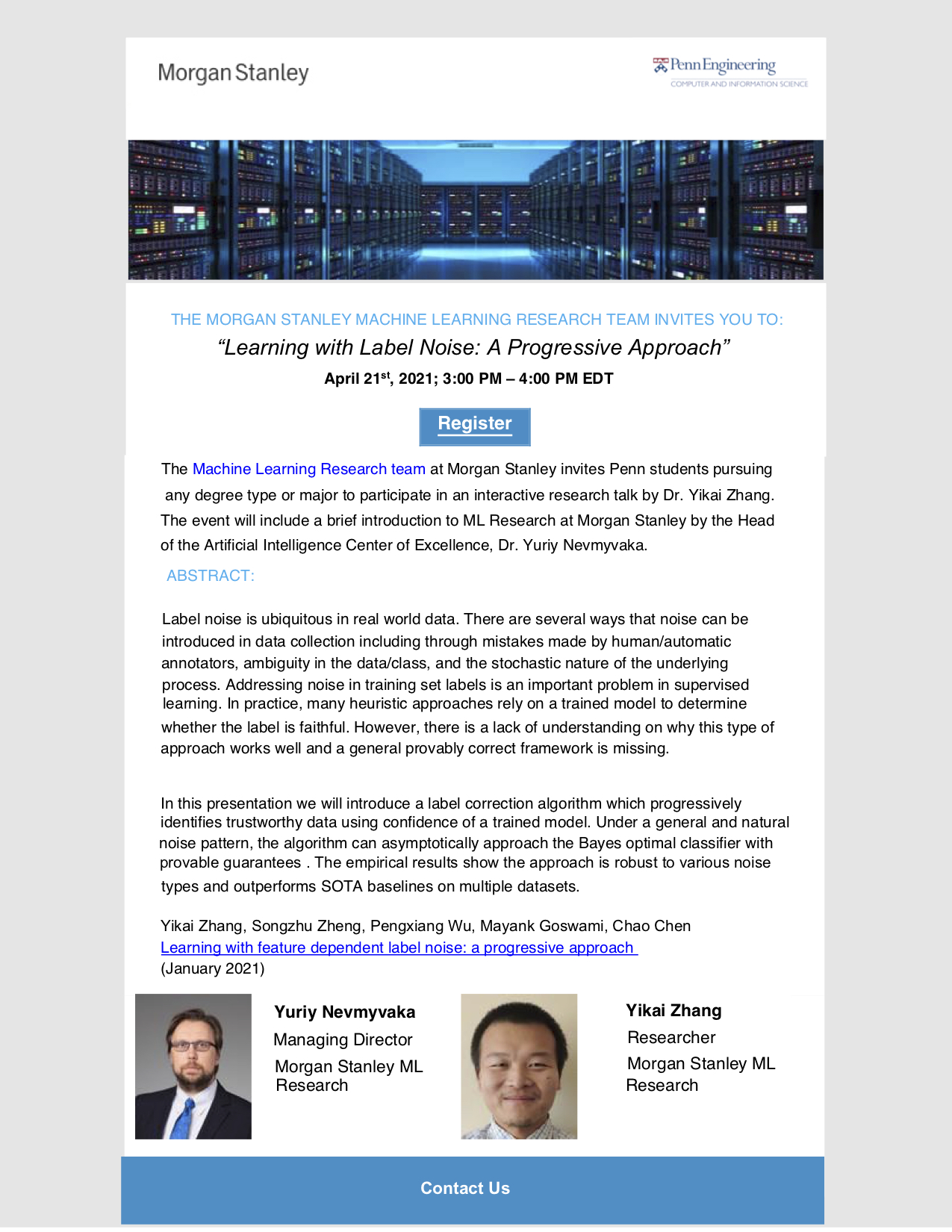
The Machine Learning Research team at Morgan Stanley invites Penn students pursuing any degree type or major to participate in an interactive research talk by Dr. Yikai Zhang. The event will include a brief introduction to ML Research at Morgan Stanley by the Head of the Machine Learning Center of Excellence, Dr. Yuriy Nevmyvaka.
ABSTRACT:
Label noise is ubiquitous in real world data. There are several ways that noise can be introduced in data collection including through mistakes made by human/automatic annotators, ambiguity in the data/class, and the stochastic nature of the underlying process. Addressing noise in training set labels is an important problem in supervised learning. In practice, many heuristic approaches rely on a trained model to determine whether the label is faithful. However, there is a lack of understanding on why this type of approach works well and a general provably correct framework is missing.
In this presentation we will introduce a label correction algorithm which progressively identifies trustworthy data using confidence of a trained model. Under a general and natural noise pattern, the algorithm can asymptotically approach the Bayes optimal classifier with provable guarantees. The empirical results show the approach is robust to various noise types and outperforms SOTA baselines on multiple datasets.
Yikai Zhang, Songzhu Zheng, Pengxiang Wu, Mayank Goswami, Chao Chen
Learning with feature dependent label noise: a progressive approach
(January 2021)
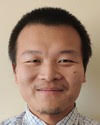