
- This event has passed.
CIS Seminar: ” Exploring the role of scientific machine learning in electric power system decarbonization”
December 8, 2022 at 3:30 PM - 4:30 PM
Electric power systems lie at the heart of efforts to mitigate and adapt to the effects of climate change. Mitigation requires shifting electricity generation away from carbon-emitting technologies toward zero carbon sources such as wind and solar generation, and converting energy end uses like transportation and space conditioning to use that electricity. Adaptation requires designing electricity infrastructure to withstand extreme weather conditions, including heat waves, hurricanes, and wildfire. Computing and data sit at the heart of planning for these changes so that they are cost effective and reliable. This talk will survey three challenges in this space, namely, (i) ensuring power system stability in the face of massive changes in generation infrastructure, (ii) exploring the scope of infrastructure change required to reliably serve millions of electrified cars and buildings and (iii) quantifying the risk of wildfire caused by millions of pieces of electricity infrastructure, and the risk reduction achieved by a suite of possible upgrades to that infrastructure. In the course of the survey, the talk will discuss how machine learning tools — including state of the art scientific machine learning principles — can be brought to bear on these problems.
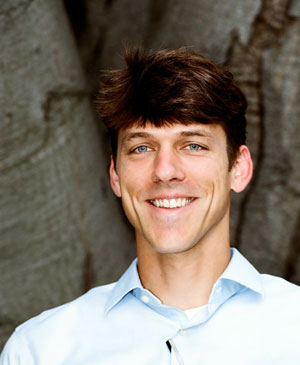
Duncan Callaway
Associate Professor of Energy and Resources at the University of California, Berkeley
Duncan Callaway is an Associate Professor of Energy and Resources at the University of California, Berkeley. He is also a faculty affiliate in Electrical Engineering and Computer Science, and a faculty scientist at Lawrence Berkeley Laboratory. He received his PhD from Cornell University. He has held engineering positions at Davis Energy Group and PowerLight Corporation, and academic positions at UC Davis, the University of Michigan and UC Berkeley. Duncan teaches courses on electric power systems and at the intersection of statistical learning and energy. His research focuses on grid integration of renewable electricity, and models and control strategies for power system dynamics, demand response, electric vehicles and electricity storage.