
- This event has passed.
CIS Seminar: “Diffusion Models in Computer Vision”
November 30, 2023 at 3:30 PM - 4:30 PM
Denoising diffusion models represent a recent emerging topic in computer vision, demonstrating impressive results in generative modeling. A diffusion model is a deep generative model that is based on two stages, a forward diffusion stage and a reverse diffusion stage. In the forward diffusion stage, the input data is gradually perturbed over several steps by adding Gaussian noise. In the reverse stage, a model is tasked at recovering the original input data by learning to gradually reverse the diffusion. Diffusion models are widely appreciated for the quality and diversity of the generated images. In this talk I will present our recent work on how diffusion models can be employed for solving computer vision problems. First, I will discuss temporal action segmentation for comprehending human behaviors in complex videos, which aims to process a long video and produce a sequence that delineates the action category for each frame. I will present a framework based on the denoising diffusion model that iteratively produces action predictions starting with random noise, conditioned on the features of the input video. To effectively capture three key characteristics of human actions, namely the position prior, the boundary ambiguity, and the relational dependency, we propose a cohesive masking strategy for the conditioning features. Next, I will briefly discuss how diffusion models are employed to solve the problems of person image synthesis, cloth-changing person re-identification, and limited field of view cross-view geo-localization and present state of results.
Although the use of diffusion models has yielded positive results in text-to-image generation, there is a notable lack of research regarding the understanding of these models. For example, there is a rising need to understand how to design effective prompts that produce the desired outcome. Next, I will briefly talk about our ongoing work on Reverse Stable Diffusion: What prompt was used to generate this image? I will end this talk by briefly discussing our recent work that underscores the significance of incorporating symmetries into diffusion models, by enforcing equivariance to a general set of transformations within DDPM’s reverse denoising learning process.
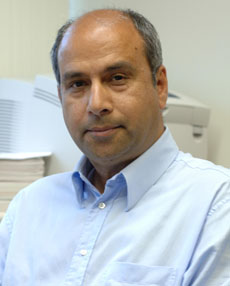
Dr. Mubarak Shah
UCF Trustee Chair Professor and founding director of Center for Research in Computer Visions at University of Central Florida
Dr. Mubarak Shah, the UCF Trustee Chair Professor, is the founding director of Center for Research in Computer Visions at University of Central Florida (UCF). Dr. Shah is a fellow of ACM, IEEE, AAAS, NAI, IAPR, AAIA and SPIE. He has published extensively on topics related to human activity and action recognition, visual tracking, geo registration, visual crowd analysis, object detection and categorization, shape from shading, etc. He has served as ACM and IEEE Distinguished Visitor Program speaker. He is a recipient of 2022 PAMI Mark Everingham Prize for pioneering human action recognition datasets; 2019 ACM SIGMM Technical Achievement award; 2020 ACM SIGMM Test of Time Honorable Mention Award for his paper “Visual attention detection in video sequences using spatiotemporal cues”; 2020 International Conference on Pattern Recognition (ICPR) Best Scientific Paper Award; an honorable mention for the ICCV 2005 Where Am I? Challenge Problem; 2013 NGA Best Research Poster Presentation; 2nd place in Grand Challenge at the ACM Multimedia 2013 conference; and runner up for the best paper award in ACM Multimedia Conference in 2005 and 2010. At UCF he has received Pegasus Professor Award; University Distinguished Research Award; Faculty Excellence in Mentoring Doctoral Students; Scholarship of Teaching and Learning award; Teaching Incentive Program award; Research Incentive Award.