
- This event has passed.
CBE Seminar: “Structure-Independent Peptide Binder Design via Generative Language Models” (Chatterjee, Duke University)
November 1, 2023 at 3:30 PM - 4:30 PM
The ability to modulate pathogenic proteins represents a powerful treatment strategy for diseases. Unfortunately, many proteins are considered “undruggable” by small molecules, and are often intrinsically disordered, precluding the usage of structure-based tools for binder design. To address these challenges, we have developed a suite of algorithms that enable the design of target-specific peptides via protein language model embeddings, without the requirement of 3D structures. First, we train a model, SaLT&PepPR, that leverages ESM-2 embeddings to efficiently select high-affinity peptides from natural protein interaction interfaces. Next, we develop a generator-discriminator model, PepPrCLIP, based on the CLIP architecture, to generate and screen de novo peptides with selectivity to a specified target protein. As input to the discriminator, we create a Gaussian diffusion generator to sample an ESM-2 based latent space, fine-tuned on experimentally-valid peptide sequences. Finally, to enable target-conditioned de novo generation of binding peptides, we train a masked language model, PepMLM to discontinuously unmask peptides given target sequences. Our final model demonstrates low perplexities across both existing and generated peptide sequences. We experimentally fuse model-derived peptides to E3 ubiquitin ligase domains and reliably identify candidates exhibiting functionally potent degradation of undruggable, disordered targets in cancer models. Overall, our work enables generation of programmable modulators to any target protein, without the requirement of conformationally stable three-dimensional structures.
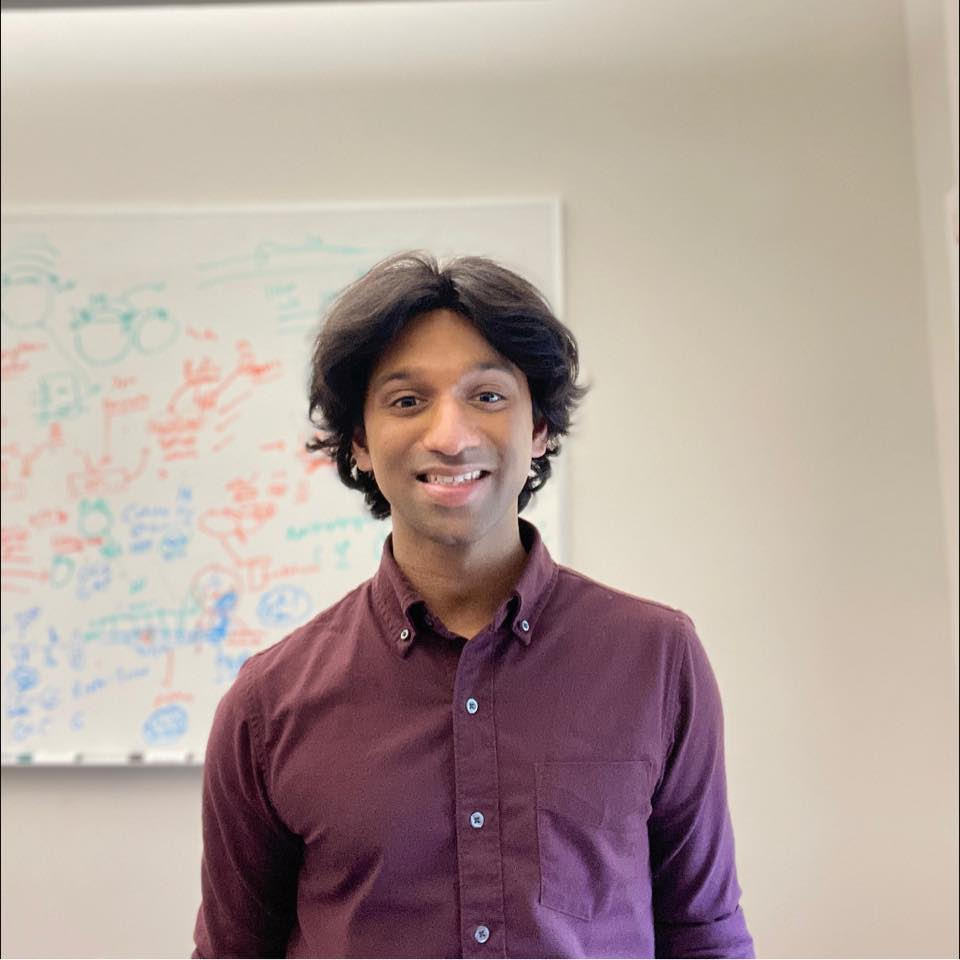
Pranam Chatterjee
Assistant Professor
Pranam Chatterjee is an Assistant Professor of Biomedical Engineering and Computer Science at Duke University. Research in his Programmable Biology Group exists at the interface of computational design and experimental engineering, specifically employing artificial intelligence (AI) to generate programmable proteins for applications in genome, proteome, and cell engineering. Having completed his SB, SM, and PhD from MIT, he has engineered genome editing technologies that represent some of the broadest, safest, and most effective CRISPR enzymes to date. More recently, his research at Duke has extended to the emergent field of “proteome” editing, where his team leverages generative language models to design potent “guides” peptides that bind and post-translationally modify pathogenic proteins, including those implicated in genetic diseases, viral diseases, and cancer. His established expertise in deep learning-based design are further being applied to develop transcription factor-based stem cell differentiation protocols for ovarian cell types, including primordial germ cells and oocytes. Overall, the long-term goals of his lab are to de novo design protein-based therapeutics by integrating the newest advances in generative AI with robust experimental engineering platforms.