
- This event has passed.
CBE Seminar: “No Equations, No Variables, No Space, No Time: Data and the Modeling of Complex Systems”
September 8, 2021 at 3:30 PM - 4:30 PM
Abstract
Obtaining predictive dynamical equations from data lies at the heart of science and engineering modeling, and is the linchpin of our technology. In mathematical modeling, one typically progresses from observations of the world (and some serious thinking!) first to equations for a model, and then to the analysis of the model to make predictions. Good mathematical models give good predictions (and inaccurate ones do not), but the computational tools for analyzing them are the same: algorithms that are typically based on closed form equations.
While the skeleton of the process remains the same, today we witness the development of mathematical techniques that operate directly on observations data, and appear to circumvent the serious thinking that goes into selecting variables and parameters and deriving accurate equations. The process then may appear to the user a little like making predictions by “looking in a crystal ball”. Yet the “serious thinking” is still there and uses the same – and some new – mathematics: it goes into building algorithms that jump directly from data to the analysis of the model (which is now not available in closed form) so as to make predictions. Our work here presents a couple of efforts that illustrate this ”new” path from data to predictions. It really is the same old path, but it is traveled by new means.
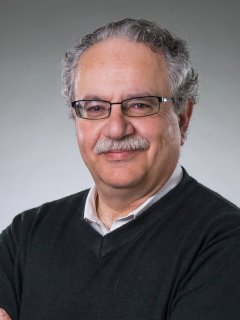
Yannis Kevrekidis, PhD
Bloomberg Distinguished Professor, Chemical and Biomolecular Engineering - Johns Hopkins University
Yannis Kevrekidis, Bloomberg Distinguished Professor in the departments of Chemical and Biomolecular Engineering and Applied Mathematics and Statistics, and in the School of Medicine’s Department of Urology at Johns Hopkins University, pioneered the approach known as “equation-free computation.” Kevrekidis’ research interests have always centered around the dynamic behavior of physical, chemical, and biological processes; the types of instabilities they exhibit; the patterns they form; and their computational study. More recently, he has developed an interest in multiscale computations and the modeling of complex systems. Along with several students and collaborators, he developed what he calls the “equation-free” approach to complex systems modeling, explored its capabilities in several areas, and is now working on linking it with modern data mining/machine learning techniques in what could be called an “equation-free and variable-free” approach.
Kevrekidis earned a bachelor’s degree in chemical engineering at the National Technical University in Athens and a PhD at the University of Minnesota’s Department of Chemical Engineering and Materials Science. He arrived at Johns Hopkins in 2017 after serving as the Pomeroy and Betty Perry Smith Professor in Engineering at Princeton University, where he was professor of Chemical and Biological Engineering, senior faculty in Applied and Computational Mathematics, and associate faculty member in Mathematics.