
- This event has passed.
ASSET Seminar: “Wood Wide Models”
October 9, 2024 at 12:00 PM - 1:15 PM
Abstract:
Foundation models are monolithic models that are trained on a broad set of data, and which are then in principle fine-tuned to various specific tasks. But they are ill-suited to many heterogeneous settings, for instance numeric tabular data, or numeric time-series data, where training a single monolithic model over a large collection of such datasets is not meaningful. For instance, why should numeric times series of stock prices have anything to do with time series comprising the vital signs of an ICU patient? For such settings, we propose the class of wood wide models.
The wood wide web is often used to describe an underground network of fungal threads that connect many trees and plants together, which stands in contrast to a large concrete foundation on top of which we might build specialized buildings. Analogously, in contrast to a single foundation model upon which one might build specialized models, we can have many smaller wood wide models that all borrow subtler ingredients from each other. But to be able to share nutrients from the wood wide web, trees need a special root based architecture that can connect to these fungal threads. Accordingly, to operationalize wood wide models, we develop a novel neuro-symbolic architecture, that we term “neuro-causal”, that uses a synthesis of deep neural models and causal graphical models to automatically infer higher level symbolic information from lower level “raw features”, while also allowing for rich relationships among the symbolic variables. Neuro-causal models retain the flexibility of modern deep neural network architectures while simultaneously capturing statistical semantics such as identifiability and causality, which are important to discuss ideal, target representations and their tradeoffs. But most interestingly, these can further form a web of wood wide models when they borrow in part from a shared conceptual ontology, as well as causal mechanisms. We provide conditions under which this entire architecture can be recovered uniquely. We also discuss efficient algorithms and provide experiments illustrating the algorithms in practice.
Zoom Link (if unable to attend in-person): https://upenn.zoom.us/j/98029108883
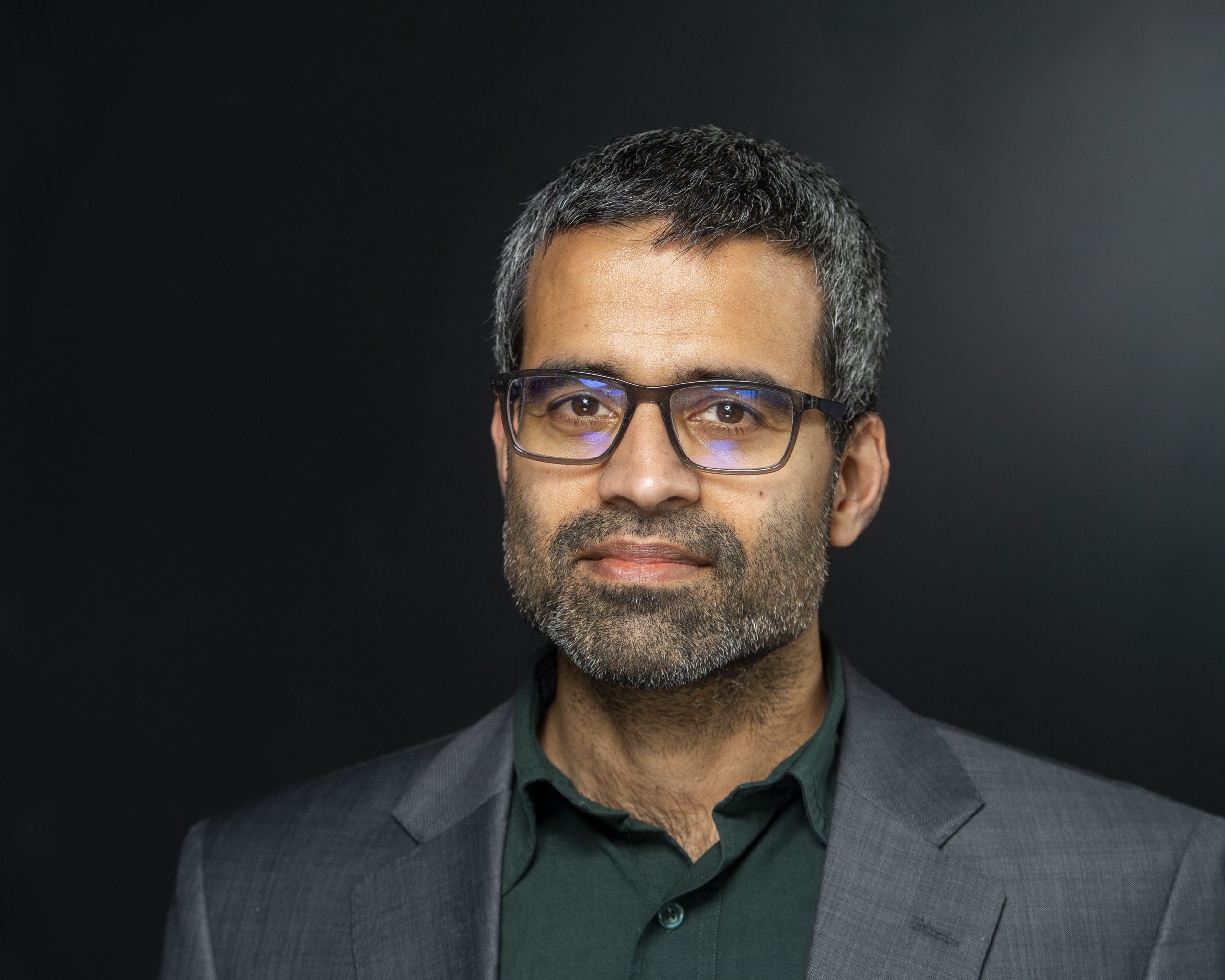
Pradeep Ravikumar
Professor
Pradeep Ravikumar is a Professor in the Machine Learning Department, School of Computer Science at Carnegie Mellon University. He is a Sloan Fellow, a Siebel Scholar, a recipient of the NSF CAREER Award, and a co-editor-chief of the Journal of Machine Learning Research. He was previously the Associate Editor-in-Chief for IEEE Transactions on Pattern Analysis and Machine Intelligence (TPAMI). His recent research interests are in neuro-symbolic AI, combining statistical machine learning, and symbolic and causal learning.