
- This event has passed.
ASSET Seminar: “Towards Improving the Reliability of AI: Perspectives from Uncertainty Quantification and Fairness”
January 22 at 12:00 PM - 1:15 PM
Abstract:
Artificial intelligence holds enormous promise to automate our life. At the same time, AI systems can be incredibly brittle, unreliable, and biased. In this talk, I will present a few approaches towards making AI more reliable, trustworthy, and fair, drawing on recent work on uncertainty quantification and algorithmic fairness. Specifically, I will discuss how to make large language models more calibrated, how to construct prediction sets for data with multiple missing outcomes, and how to obtain optimally accurate fair classifiers under constraints on linear disparity measures.
Zoom Link (if unable to attend in-person): https://upenn.zoom.us/j/97670810812
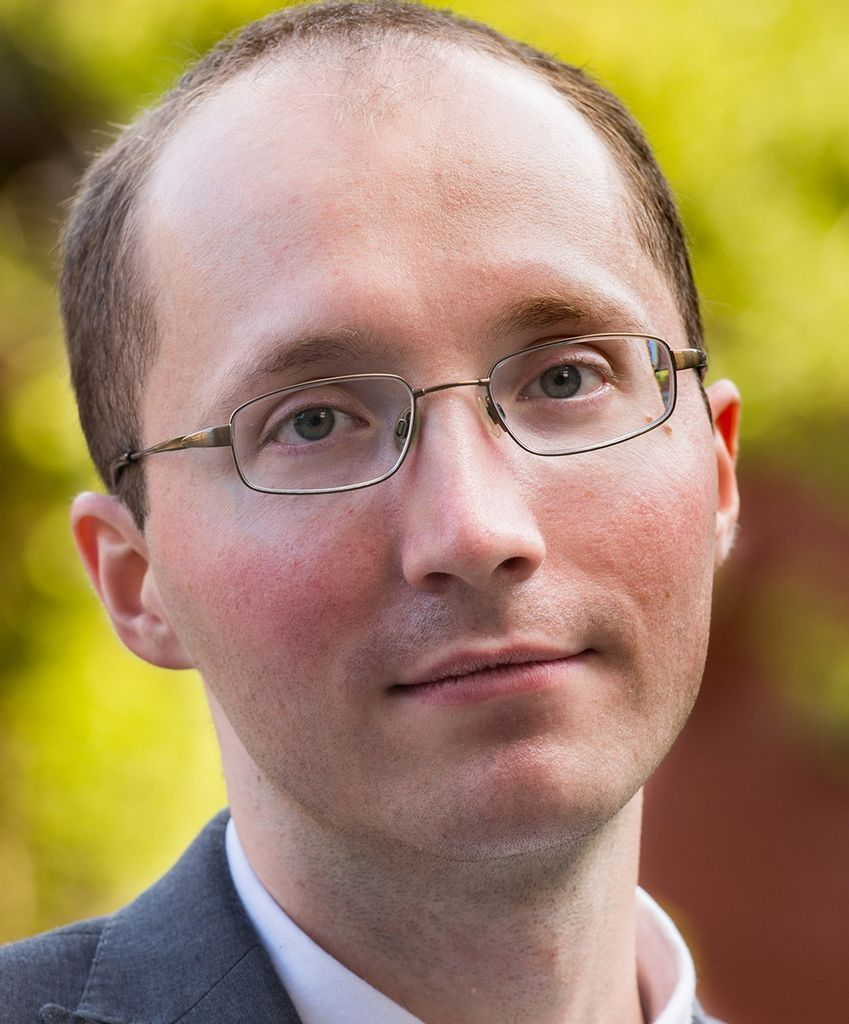
Edgar Dobriban
Associate Professor
Edgar Dobriban is an associate professor in the Department of Statistics and Data Science at the University of Pennsylvania, with a secondary appointment in Computer and Information Science. He obtained a PhD in statistics from Stanford University in 2017. His research is at the interface of statistics, machine learning, and artificial intelligence.
He received the Peter Gavin Hall Early Career Prize from the Institute of Mathematical Statistics (IMS) in 2024, the Noether Early Career Scholar Award in Non-Parametric Statistics from the American Statistical Association (ASA) in 2024, an Emerging Leader Award from the Committee of Presidents of Statistical Societies (COPSS) in 2023, a Sloan Research Fellowship in Mathematics in 2023, a Bernoulli Society New Researcher Award in 2023, and a U.S. National Science Foundation CAREER award in 2021.