
- This event has passed.
MEAM Ph.D. Thesis Defense: “Data-Driven Model Discovery for Non-Equilibrium Phenomena: Unraveling Continuum Behavior from Stochastic Dynamics”
July 10, 2023 at 10:00 AM - 11:00 AM
Non-equilibrium phenomena are ubiquitous across material systems and of great technological relevance. Examples of such phenomena include diffusion processes in liquid and gases, viscoelasticity and plasticity in solids, and rheological behavior of colloidal and granular media. Despite their ubiquity and importance, the understanding of non-equilibrium phenomena remains in its infancy compared with classical equilibrium thermodynamics and statistical mechanics from both theoretical and computational aspects. As a consequence, current modeling and simulation strategies, including multiscale paradigms, are mostly trapped within a compromise between computational efficiency and physical fidelity.
This thesis leverages recent advances in non-equilibrium physics, together with emerging machine learning techniques, to develop theoretical and computational paradigms for learning continuum evolution equations using data-driven methods. First, we present a new strategy for continuum model discovery that uses fluctuation theorems, particularly, the Jarzynski equality, to identify the reversible (elastic) and irreversible (dissipative) response. Second, we propose a machine learning architecture called Variational Onsager Neural Networks (VONNs) to learn thermodynamically consistent non-equilibrium evolution PDEs based on Onsager’s variational principle. Thirdly, we develop a multiscale machine learning framework called Statistical-Physics-Informed Neural Networks (Stat-PINNs) to uniquely determine coarse-grained dissipative evolution equations from stochastic particle dynamics by leveraging fluctuation-dissipation relations. Lastly, we introduce a statistical mechanics framework with quantified uncertainty to extrapolate material behavior to different loading conditions (including far-from-equilibrium conditions) or material systems.
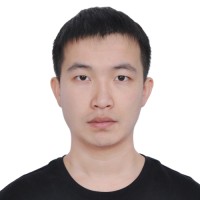
Shenglin Huang
Ph.D. Candidate, Department of Mechanical Engineering & Applied Mechanics, University of Pennsylvania
Advisor: Celia Reina