
- This event has passed.
MEAM Seminar: “Disease Indicator Prediction in Vascular Flows via Physics-Informed Deep Learning”
August 23, 2022 at 10:00 AM - 11:30 AM
Unlike diseases with strong genetic predisposition, such as cancer, there exist no accurate personalized diagnostic tools for disorders such as Hypertensive Pregnancy Disorders (HPD) and its mechanism remain understudied. For the purpose of performing diagnostics, we require disease indicators (biomarkers) that are strongly correlated with the underlying condition, i.e. absolute vascular pressure for hypertension. Measuring absolute pressure requires an invasive procedure and it is impossible for small vessels and in pregnant subjects, but an informed estimate is possible via the use of Computational Fluid Dynamics. Computational Fluid Dynamics can also be employed for studying the relation between disease outcomes and biomarkers, by constructing virtual representations of a human body called Digital Twins and performing simulations for different parameters. Unfortunately the computational cost of CFD is very high thus studying the physiology of large cohorts of patients or making real time predictions is impossible. Therefore, fast surrogates that generalize across different patients and parameters are required.
In this talk, I will leverage aspects of both computational mechanics and deep learning to develop methods for predicting complex biomarkers that cannot be measured in the clinic, such as absolute pressure, for performing patient specific diagnostics. First, I will discuss the feasibility of estimating vascular parameters for assessing Hypertensive Pregnancy Disorders using a combination of Bayesian inference and a reduced order Navier-Stokes model and show that despite the accuracy of this procedure, its computational complexity makes it impossible to be employed in clinical practice. Second, I will discuss leveraging Physics-Informed Neural Networks (PINNs) for designing Deep Learning surrogates to alleviate computational drawbacks of the above pipeline for one patient. Third, I will discuss machine learning inference in function spaces, called Operator Learning, and more specifically the LOCA method and the potential of online biomarker predictions for different patients employing operator learning on manifolds and quantifying the uncertainty of predictions.
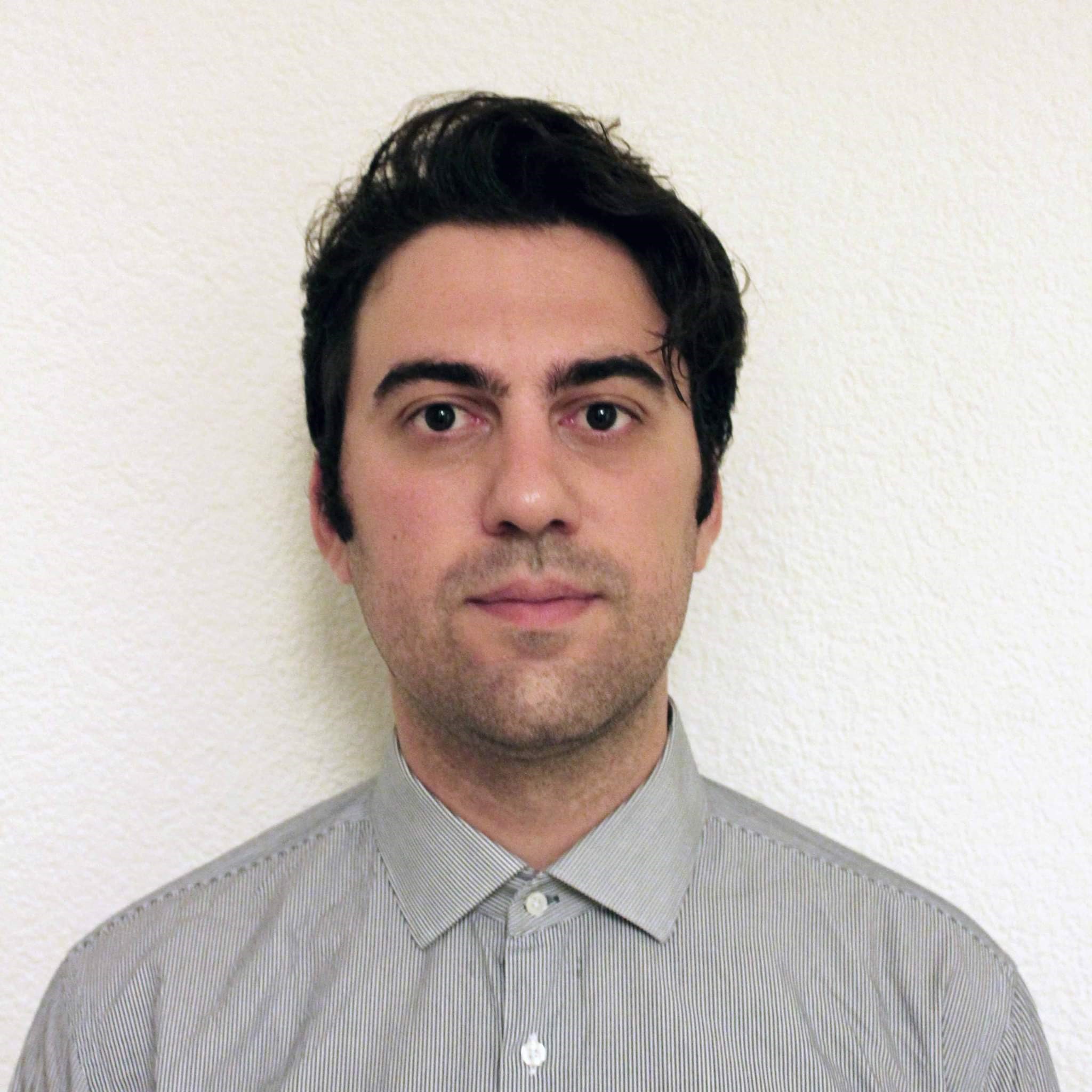
Georgios Kissas
Ph.D. Candidate, Department of Mechanical Engineering & Applied Mechanics, University of Pennsylvania
Advisor: Paris Perdikaris