
- This event has passed.
MEAM Seminar: “Bridging Physical Models and Observational Data with Physics-informed Deep Learning”
December 8, 2020 at 10:30 AM - 12:00 PM
Physical models of many natural and engineered systems are, at best, only partially known; a common setting under which classical analytical or computational tools inevitably face challenges and introduce many sources of uncertainty. Therefore, observational data plays a crucial role, yet our ability to collect them far outpaces our ability to sensibly assimilate it, let alone understand it. Despite their towering empirical success, machine learning approaches are not currently able to extract interpretable information and knowledge from this data deluge. Moreover, purely data-driven methods may fit observations very well, but predictions may be physically inconsistent or implausible, due to extrapolation or observational biases, for example. In this talk we will discuss the foundations of a new family of machine learning methods coined as physics-informed neural networks, that aim to seamlessly bridge this gap by synthesizing incomplete physics-based models with imperfect observational data. Specifically, we will illustrate the mechanisms by which deep neural networks can be constrained to respect fundamental laws of physics, but also highlight certain pathologies and limitations that arise during this process. Strikingly, some of the latter can be addressed by exploring connections to classical methods in numerical analysis and optimization, opening the path to designing more principled algorithms and deep learning architectures that do not simply rely on guesswork. Finally, we will demonstrate the power of these methods across a range of diverse engineering applications, including problems in design optimization, heat transfer, wave propagation, cardiovascular fluid mechanics, and modeling of COVID-19 spread dynamics.
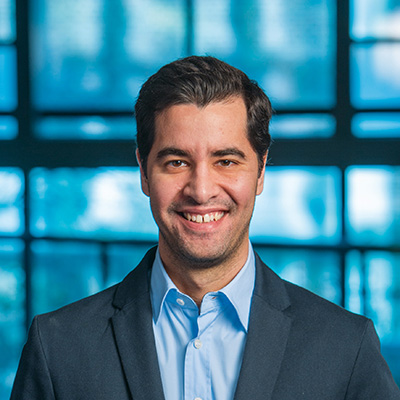
Paris Perdikaris
Assistant Professor, Department of Mechanical Engineering and Applied Mechanics, University of Pennsylvania
Paris Perdikaris is an Assistant Professor in the Department of Mechanical Engineering and Applied Mechanics at the University of Pennsylvania. He received his PhD in Applied Mathematics at Brown University in 2015, and, prior to joining Penn in 2018, he was a postdoctoral researcher at the department of Mechanical Engineering at the Massachusetts Institute of Technology working on physics-informed machine learning and design optimization under uncertainty. His work spans a wide range of areas in computational science and engineering, with a particular focus on the analysis and design of complex physical and biological systems using machine learning, stochastic modeling, computational mechanics, and high-performance computing. Current research thrusts include physics-informed machine learning, uncertainty quantification in deep learning, engineering design optimization, and data-driven non-invasive medical diagnostics. His work and service has received several distinctions including the DOE Early Career Award (2018), the AFOSR Young Investigator Award (2019), and the Ford Motor Company Award for Faculty Advising (2020).