
- This event has passed.
ESE Fall Seminar – “Non-Parametric Analysis of Dynamical Systems: From Recurrent Sets to Generalized Lyapunov and Barrier Conditions”
November 19 at 11:00 AM - 12:00 PM
This talk presents novel non-parametric methods for analyzing dynamical systems using solely trajectory data. Our critical insight is to replace the notion of invariance, a core concept in Lyapunov Theory, with the more relaxed condition of recurrence. Specifically, a set is τ-recurrent if every trajectory that starts within the set returns to it after at most τ seconds. We leverage this notion of recurrence to develop several analysis tools and algorithms to study dynamical systems. Firstly, we consider the problem of learning an inner approximation of the region of attraction (ROA) of an asymptotically stable equilibrium point using trajectory data. We show that a τ-recurrent set containing a stable equilibrium must be a subset of its ROA under mild assumptions and develop algorithms that compute inner approximations of the ROA using counter-examples of recurrence obtained by sampling finite-length trajectories. Secondly, we generalize Lyapunov and Barrier Function Methods to allow for non-monotonic evolution of the function values by only requiring sub-level sets to be τ-recurrent (instead of invariant). We provide conditions for stability and safety using τ-monotonic functions (functions whose value along trajectories monotonically increases or decreases after at most τ seconds) and develop a verification algorithm that leverages GPU parallel processing power to verify stability and safety using only trajectory information. We finalize by discussing future research directions and possible extensions for control.
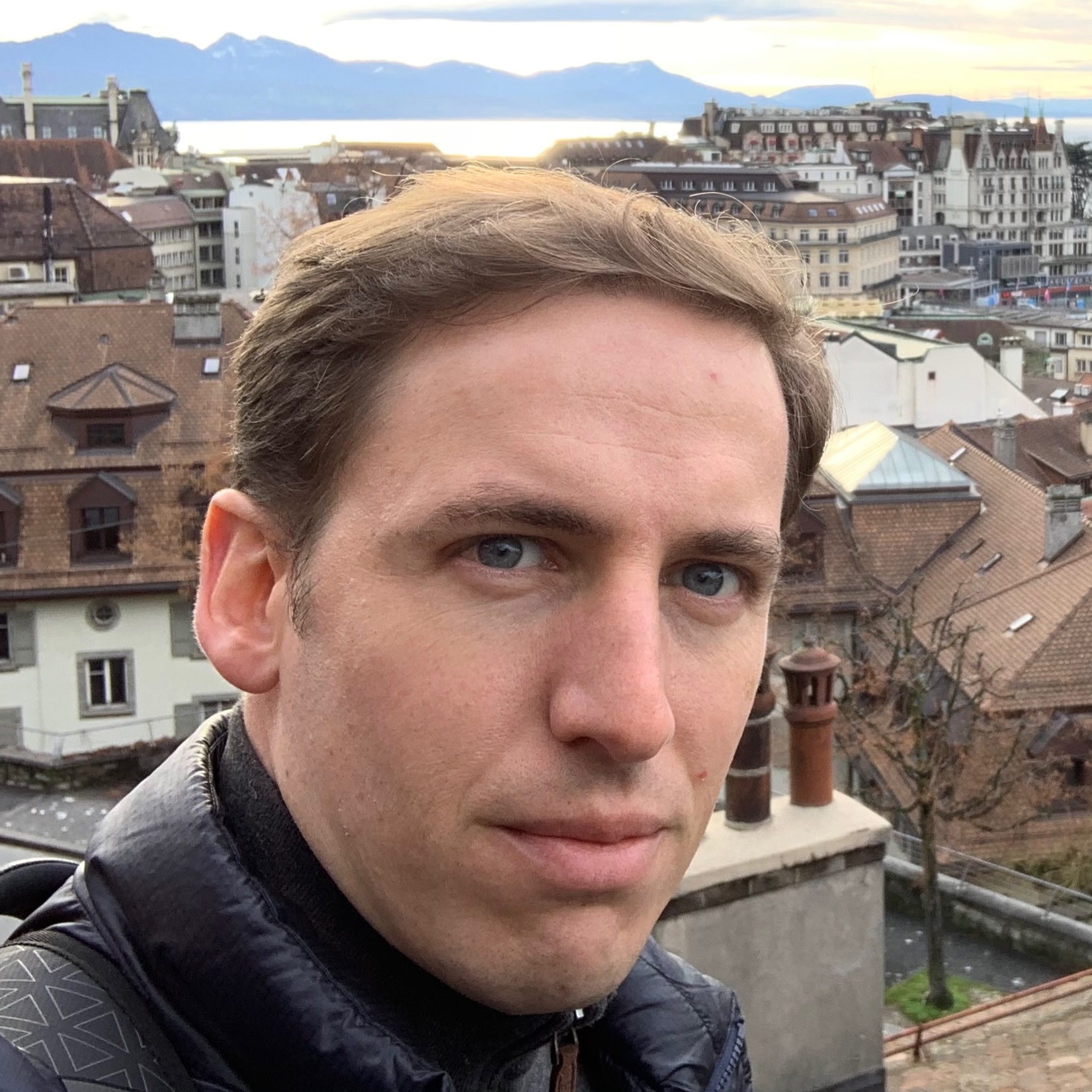
Enrique Mallada
Associate Professor of Electrical and Computer Engineering, Johns Hopkins University
Enrique Mallada has been an Associate Professor of Electrical and Computer Engineering at Johns Hopkins University since 2022. Before joining Hopkins in 2016, he was a Post-Doctoral Fellow in the Center for the Mathematics of Information at Caltech from 2014 to 2016. He received his Ingeniero en Telecomunicaciones degree from Universidad ORT, Uruguay, in 2005 and his Ph.D. in Electrical and Computer Engineering with a minor in Applied Mathematics from Cornell University in 2014. Dr. Mallada has received the Johns Hopkins Alumni Association Teaching Award in 2021, the NSF CAREER award in 2018, the Center for the Mathematics of Information (CMI) Fellowship from Caltech in 2014, and the Cornell ECE Director’s Ph.D. Thesis Research Award in 2014. His research interests lie in control and dynamical systems, machine learning, and optimization, with applications to safety-critical networks and systems.